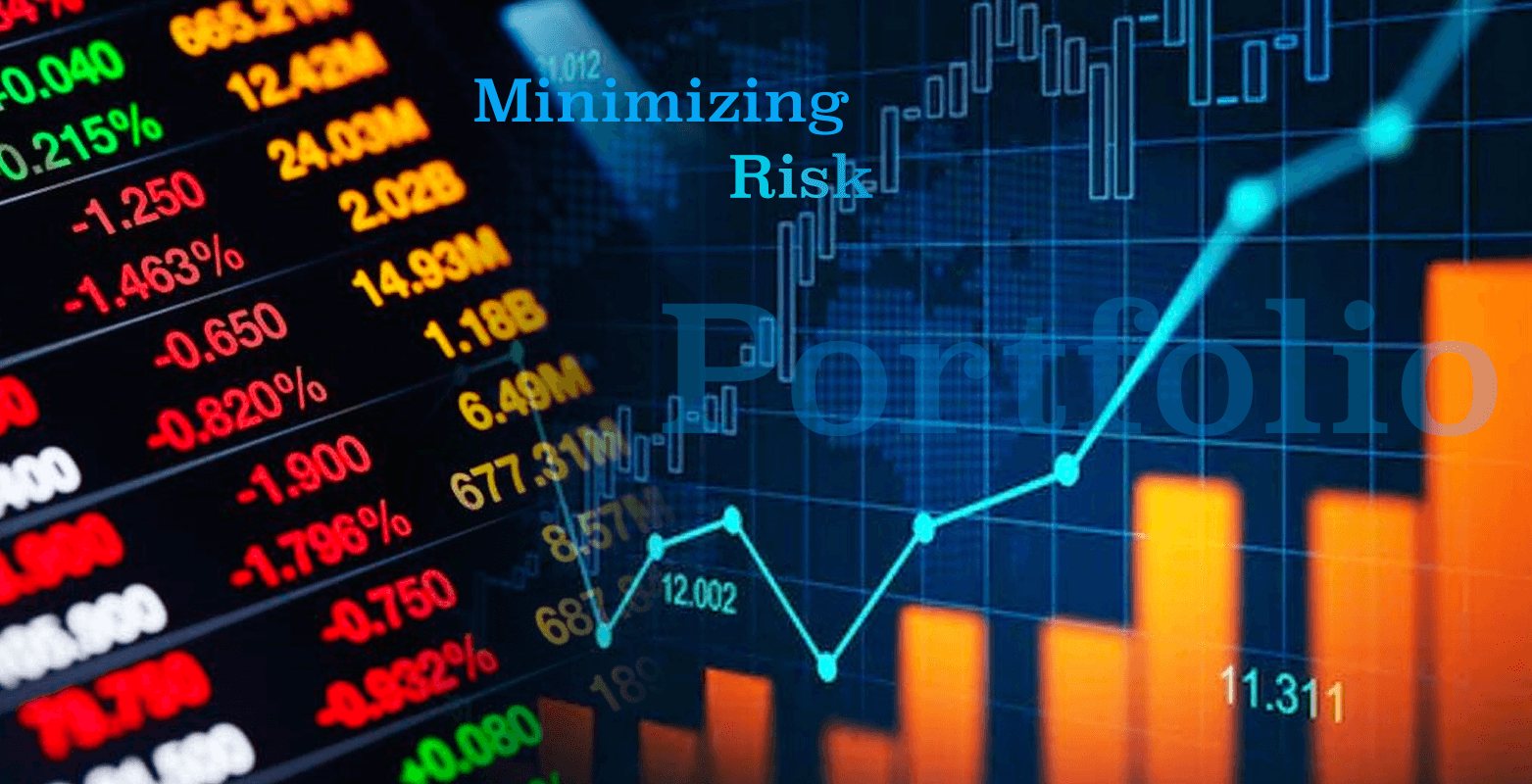
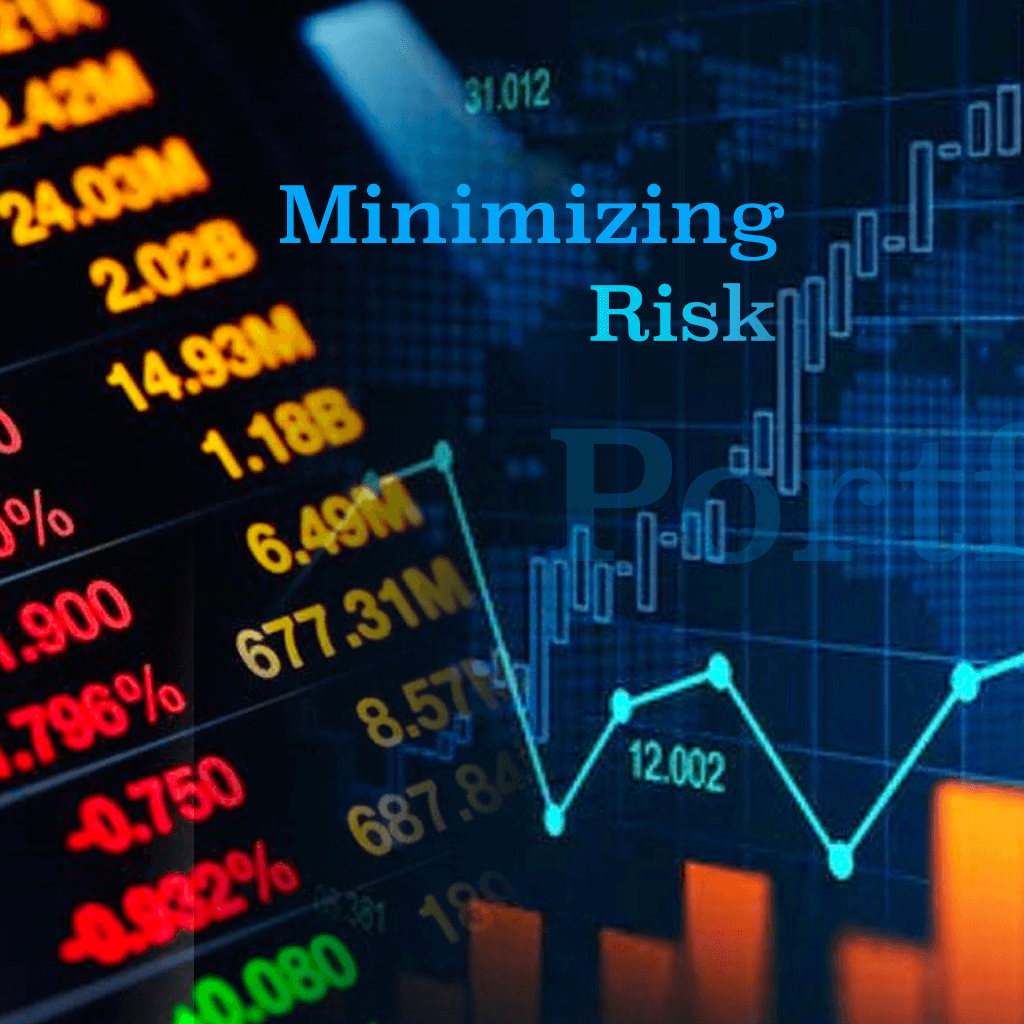
Introduction:
Warren Buffett once said, 'Risk comes from not knowing what you're doing.' In the ever-evolving landscape of finance, this statement holds truer than ever. As we delve into the intersection of finance and technology, the integration of machine learning in portfolio management emerges as a beacon of insight, promising not just returns, but a profound understanding of risk. How can the marriage of finance and artificial intelligence pave the way for optimal investment strategies? Let's navigate the complexities together and explore the transformative potential of Machine Learning in Portfolio Management.
The Evolution of Portfolio Management:
Traditional portfolio management, with its reliance on historical data and statistical models, has its limitations. In a world where market dynamics shift with unprecedented speed, the need for a more adaptive and predictive approach is evident. This is where machine learning steps in, armed with the ability to analyse vast datasets, identify patterns, and make data-driven decisions in real time.
Understanding the Landscape:
Before we dive into the technical intricacies, let's grasp the fundamental shift that machine learning brings to portfolio management. It's not just about crunching numbers; it's about leveraging algorithms that can learn, adapt, and optimise investment strategies. The goal? Enhancing returns while keeping risk at bay.
Machine Learning Models in Portfolio Management:
Predictive Analytics and Forecasting:
Machine learning models can analyse historical market data to make accurate predictions about future trends. This predictive analytics approach aids in identifying potential opportunities and risks, allowing for proactive decision-making.
Risk Management and Optimization:
Advanced machine learning algorithms excel in risk management by identifying potential pitfalls and suggesting optimal portfolio allocations. This dynamic risk optimization ensures a balance between returns and acceptable risk levels.
Sentiment Analysis for Market Trends:
Natural Language Processing (NLP) algorithms can analyse financial news, social media, and other textual data to gauge market sentiment. This sentiment analysis provides valuable insights into potential market movements, helping investors stay ahead of trends.
Implementing Machine Learning Strategies:
Now that we've scratched the surface, how does one go about implementing machine learning strategies in portfolio management? It begins with data – the lifeblood of machine learning algorithms.
Data Gathering and Cleaning:
As the saying goes, 'Garbage in, garbage out.' Quality data is paramount. Gather diverse datasets, clean them meticulously, and ensure they are free from biases or inaccuracies that could distort the learning process.
Feature Engineering:
In the world of machine learning, features are the building blocks. Identify and engineer features that have predictive power. This might include financial ratios, economic indicators, or even alternative data sources like satellite imagery or social media trends.
Model Selection and Training:
Choosing the right machine learning model is crucial. Whether it's a regression model for predicting stock prices or a classification model for risk assessment, selecting the appropriate algorithm sets the stage. Train the model on historical data to allow it to learn patterns and relationships.
Validation and Testing:
Never skip the validation step. Test the trained model on new, unseen data to ensure its predictive accuracy and generalizability. This step is crucial for preventing overfitting and ensuring the model's robustness in real-world scenarios.
Challenges in Machine Learning-Based Portfolio Management:
While the potential benefits are vast, it's important to acknowledge the challenges that come with implementing machine learning in portfolio management.
Data Privacy and Security:
Handling sensitive financial data requires stringent security measures. Ensuring compliance with data privacy regulations is non-negotiable to build trust among investors.
Interpretability of Models:
Machine learning models, especially complex ones like neural networks, can be viewed as 'black boxes.' Ensuring transparency and interpretability is essential for gaining investor confidence and regulatory compliance.
Market Dynamics and Model Adaptability:
Financial markets are dynamic, and models must adapt. The challenge lies in developing algorithms that can adjust to changing market conditions without compromising stability.
Conclusion:
In conclusion, the integration of machine learning in portfolio management heralds a new era in finance. It's a symbiotic relationship where data fuels algorithms, and algorithms empower investors. As we navigate the complexities of financial markets, let's embrace the transformative potential of machine learning to enhance returns and minimise risk. The future of portfolio management is here, driven by the intelligence of machines.
Ready to dive deeper into the world of machine learning in finance? Explore our comprehensive course at IIQF®️, where you can master the tools and techniques needed to navigate the data-driven landscape of portfolio management in 2024 and beyond.