- Fee & Course Calender
- Download Brochure
- Why Choose CPAIRM
- Faculty
- Course Outline
- Finance your Study
- Faqs
Certificate Program in AI for Risk Management (CPAIRM)
Applied AI for Risk Management
- English
-
Indian Institute of Quantitative Finance
- Book a guidance call
Quick Facts
- Program Duration
- Program Schedule
- Program Timing
- Program Start Date
CPAIRM Program | AI for Risk Management Course Highlights
The IIQF’s Super Specialization AI Program focuses on AI and ML-driven risk management and modeling use cases across various types of risks.
- Focused learning journey to cover the AI & ML based models & analytics products for risk management sub-domains.
- Insightful coverage of quantitative risk modelling use of AI & ML techniques & algorithms within BFSI & Fintech space.
- Extensive coverage of the AI & ML adoption considerations, challenges & cautions - regulatory, policy, legal, compliance and ethical.
- Practical deep-dive into AI & ML driven risk models, methodology, & mechanics across supervised, semi-supervised & unsupervised learning regimes. Coverage of best quants modelling practices and research topics in risk management domain.
- Designed to deliver know-how on BFSI risk management use-cases & applications across portfolio/credit/market/operational risk analytics.
- Wider span coverage of financial use cases encompassing risk prediction, forecasting, anomaly detection, sentiment analysis & others.
- Rigorous live classroom lectures from our expert faculty panel constituting BFSI industry subject matter experts & academic researchers
- Practical hand-on learning through Python prototyping & implementation workshops on front-to-back model building & algorithmic training exercises
- Renders technical know-how on BFSI & Fintech industry risk management application ecosystem - application architectural design & technology stack.
- BFSI industry mentor-led AI for Risk Management capstone projects and implementation white paper writing.
CPAIRM Course Outline
Quantitative Risk Management & Modelling Domain Deep Dive
Imparts domain-specific know-how on relevant application use cases
-
Portfolio Risk
- Portfolio Segmentation & Diversification
- Portfolio Optimization
- Portfolio Risk Decomposition & Attribution
- Portfolio Stress Testing & Scenario Analysis
-
Market Risk
- Asset Class Price Forecasting
- Asset Class Volatility Forecasting
- Loss or Value at Risk (VaR) Forecasting
- Market Sentiment Indicators
-
Credit Risk
- Default Risk Prediction - Probability of Default
- Recovery Risk Prediction - Loss Given Default
- Exposure Risk Prediction - Exposure At Default
-
Fraud Risk
- Anomaly Detection & Outlier Diagnostics
- Fraud Analytics
- Forensic Data Science
Statistical & AIML Models Application Use Cases
Imparts practical application of Statistical & AIML modelling methods or techniques for the specific application use-cases at hand.
-
Exploratory Data Analytics
- High Dimensional Problems & Datasets
- Big Data Mining & Manipulation
- Data Diagnostics & Inferential Analytics
- Data Augmentation
- Data Visualization & Storytelling
-
Model Development & Validation
- Regression, Classification & Clustering
- Statistical Application Use Cases
- AI & ML Application Use Cases
- Model Evaluation Metrics
- Statistical versus AI & ML Performance Comparative Analysis & Evaluation
AI & ML Adoption criteria, Considerations & Challenges
Imparts operational, strategic & technical aspects of building AI & ML in-house capability
-
AI & ML Adoption Strategy
- AI & ML Driven Credit Risk Prediction
- AI & ML Driven Market Risk Forecasting
- AI & ML Driven Fraud Risk Anomaly Detection
-
AI & ML Regulatory Strategy
- Regulatory Ask & Expectations
- Regulatory Acceptance Criteria
-
AI & ML Acceptance Strategy
- Explainable AI: AI & ML Explainability & Interpretability
- Ethical & Responsible AI - Data Privacy & Security and Model Fairness
-
AI & ML Technology Strategy
- AI & ML Infrastructure & Architecture
- AI & ML Front-To-Back Tech Stack
- AI & ML Automated Model Pipeline
Batch | Start Date | Fee | Mode | Time |
---|
Why Choose CPAIRM Program?
AI- ML Data Science Applications in Finance Podcast Series
AI- ML Data Science in Finance
Faculty
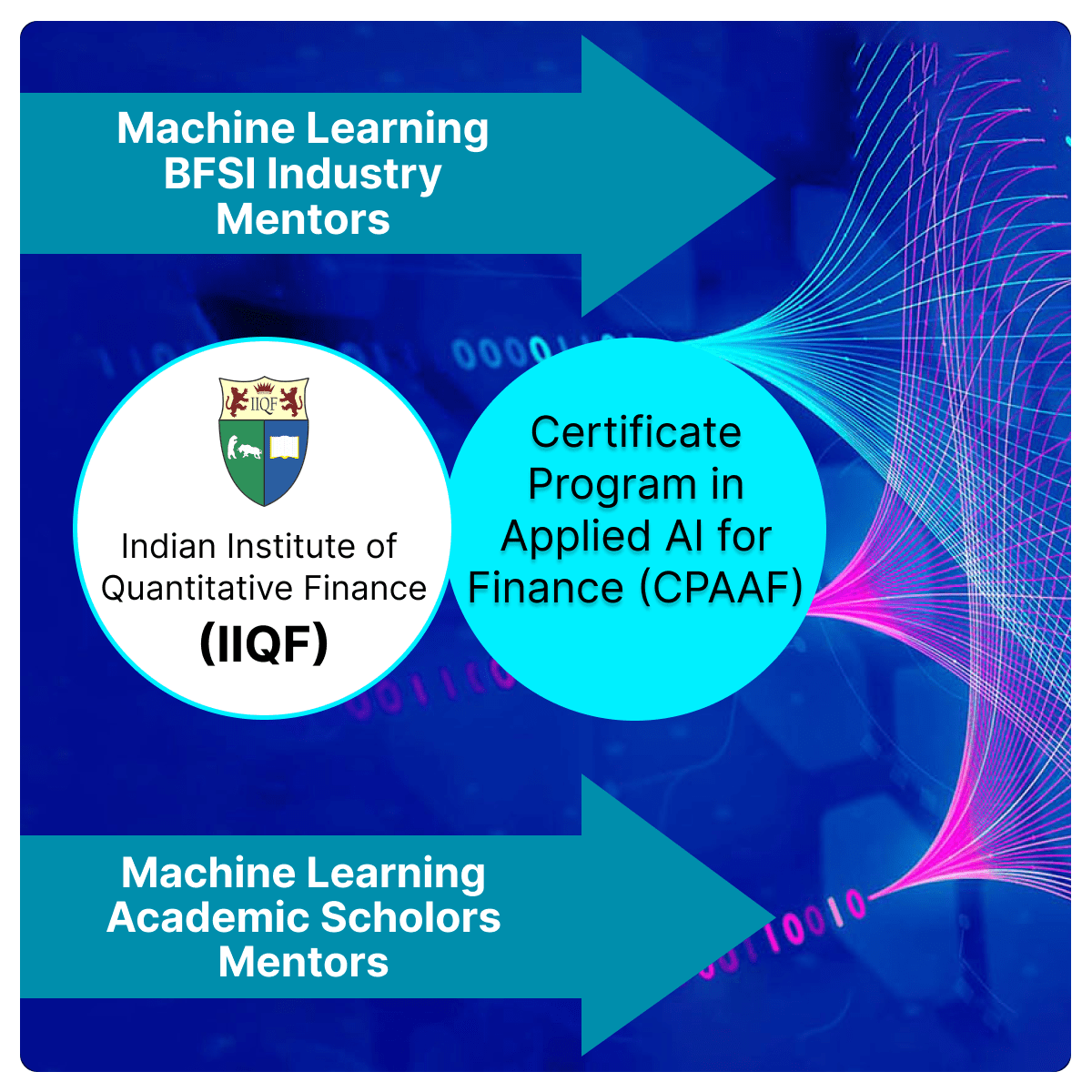
SANJAY BHATIA
Background
- Director in UBS - Risk Modelling & Analytics, Model Risk Management & Control, Chief Risk Office (CRO) Function
- MBA-Finance & MSc in Machine Learning & Artificial Intelligence from Liverpool John Moores University (LJMU)
- Post-Graduate Diploma in Machine Learning & Artificial Intelligence from IIIT-Bangalore
- Domain SME on Credit Risk , Derivatives Counterparty Credit Risk, Derivative Pricing, Stochastic Modelling, Stress Testing
ML Expertise (Teaching ML for Quantitative Finance & Risk Management)
- Financial Prediction (Regression & Classification ) - Lasso/Ridge Regression, CART Decision Trees, Ensemble Learning (Bagging & Boosting) & Support Vector Machines (SVM)
- Financial Time Series Forecasting - (Recurrent) Neural Networks, RNN-LSTM, RNN-GRU, Hybrid-RNN-LSTM-GRU
- Financial Instrument Pricing - Non-Linear & High Dimensional Derivative Pricing using Neural Networks
- ML Model Optimization – Hyperparameters Tuning K-Fold Cross-Validation, Stochastic Gradient Descent, Convergence etc.
- Regulatory & Industry ML Adoption, Challenges & Use Cases – Model Explainibility, Performance Evaluation & Testing
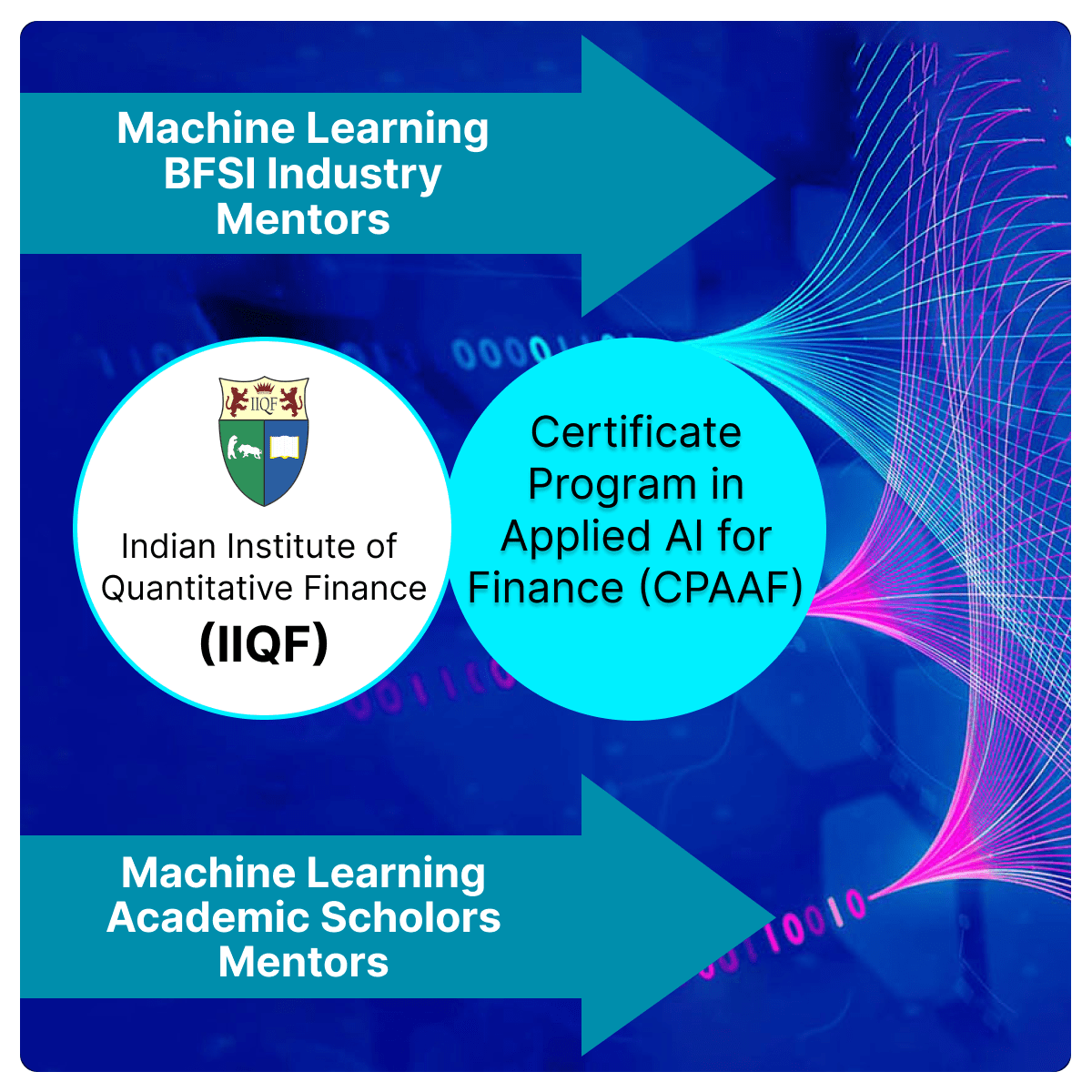
Prof Rituparna Sen
Background
- Associate Professor at the Applied Statistics Division, Indian Statistical Institute, Bangalore.
- She worked as Assistant Professor at the University of California at Davis from 2004–2011
- She has also taught courses in Chennai Mathematical Institute and Madras School of Economics.
- An elected member of the International Statistical Institute
- A council member of the International Society for Business and Industrial Statistics.
- She has been awarded the Young Statistical Scientist Award by the International Indian Statistical Association, the Best - Student Paper Award by the American Statistical Association and the Women in Mathematical Sciences award by Technical University of Munich, Germany.
ML Expertise (Teaching ML Statistics for Computational Finance)
- Authored A Book on Computational Finance with R
- Authored 30+ Research Papers & Articles
- Editor: Journal of Applied Stochastic Models in Business and Industry
- Member of CAIML (Center for Artificial Intelligence and Machine Learning) at ISI
- Associate Editor of several other journals
- Guided several masters students on theses in the ML area
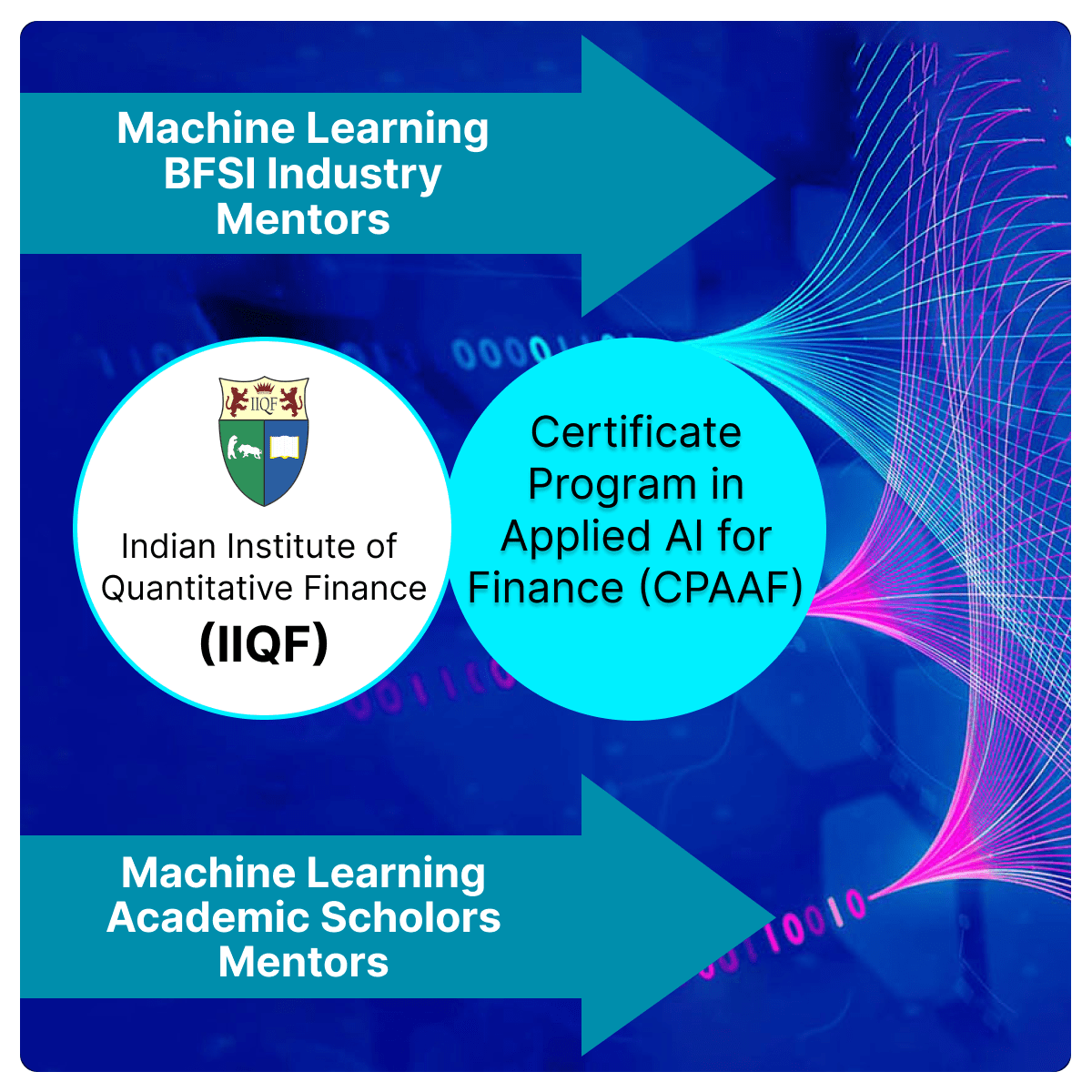
Dr. Arindam Chaudhuri
Background
- Worked as post doctoral fellow with Department of Computer Science, University of Copenhagen and Department of Computer Science, Technical University of Berlin.
- Worked as researcher with Siemens Research Labs Amsterdam and Samsung Research Labs at New Delhi & Bangalore.
- His current research interests include business analytics, artificial intelligence, machine learning, deep learning.
- He has published 4 research monographs and 60 articles in international journals and conference proceedings.
- He has served as reviewer for several international journals and conferences.
ML Expertise (Teaching ML Statistics for Computational Finance)
- Implemented Machine Learning Methods - Support Vector Machines, Artificial Neural Networks, Deep Learning Networks, Clustering, Genetic Algorithms and Evolutionary Computing with basic mathematical foundations of probability theory, fuzzy sets, rough sets, possibility theory and a variation of these for solution of various business problems.
- Integrated various artificial intelligence methods to form different soft computing frameworks such as neuro-fuzzy, fuzzy-genetic, neuro-genetic and rough-neuro-fuzzy-genetic.
- Successfully applied these methods for different categories of industrial problems such as decision theory, time series forecasting and prediction, image compression, sentiment analysis, recommendation systems, social networks analytics in order to achieve better results
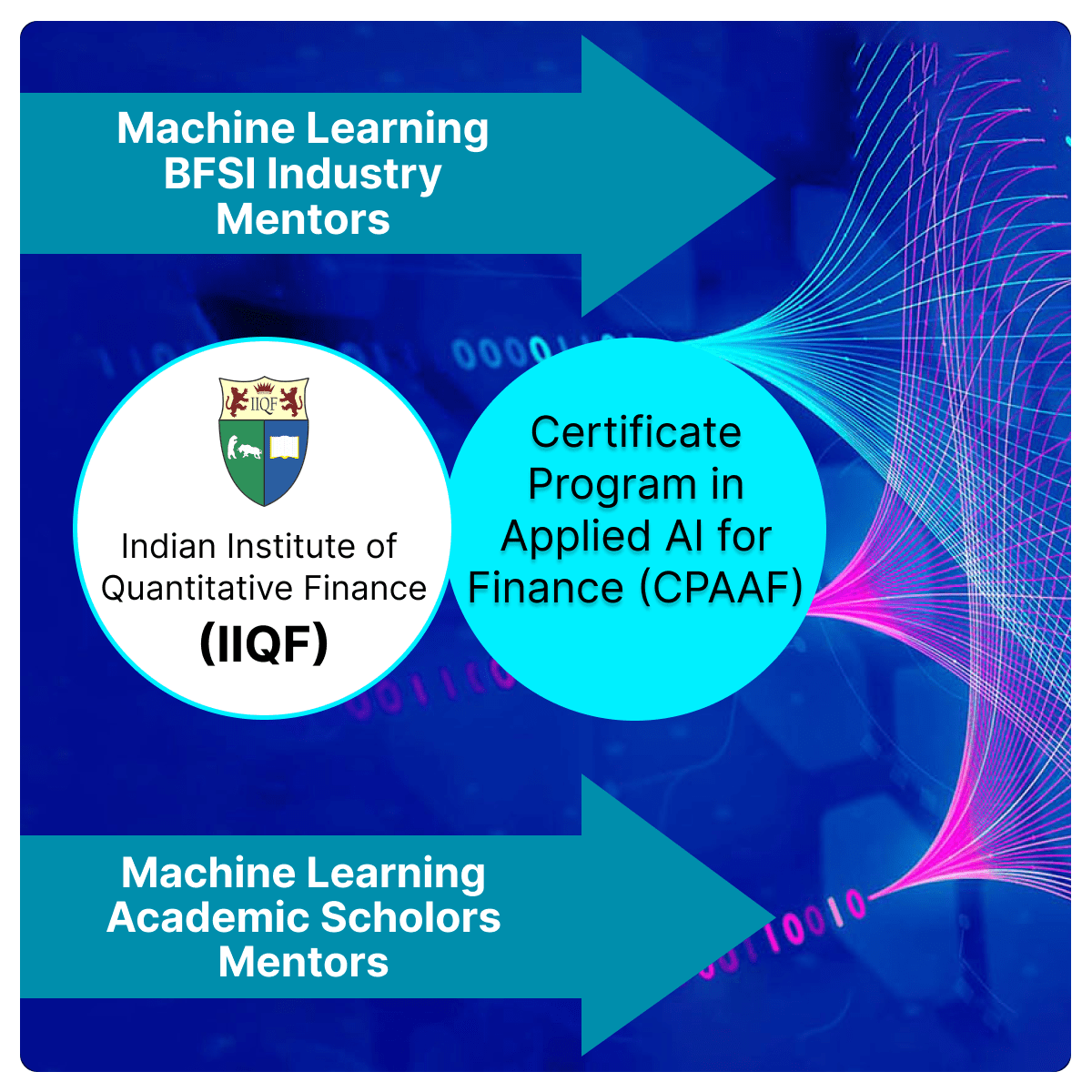
Ritesh Chandra
Background
- B. Tech. (IIT-Kanpur), PGDM (IIM-Calcutta), CFA
- 15 years banking experience in risk management with domestic and MNC banks
- Member, Board of Studies in the area of Finance at IMT-CDL, Ghaziabad
ML Expertise
- Passionate about teaching. Has been conducting workshops / training programmes for the last 8 yrs in areas of Quantitative Finance, Financial Management, Risk Management and Machine Learning
- Has contributed to a book on Applications of Blockchains in Financial Services industry
- Has worked as visiting faculty with several institutions.
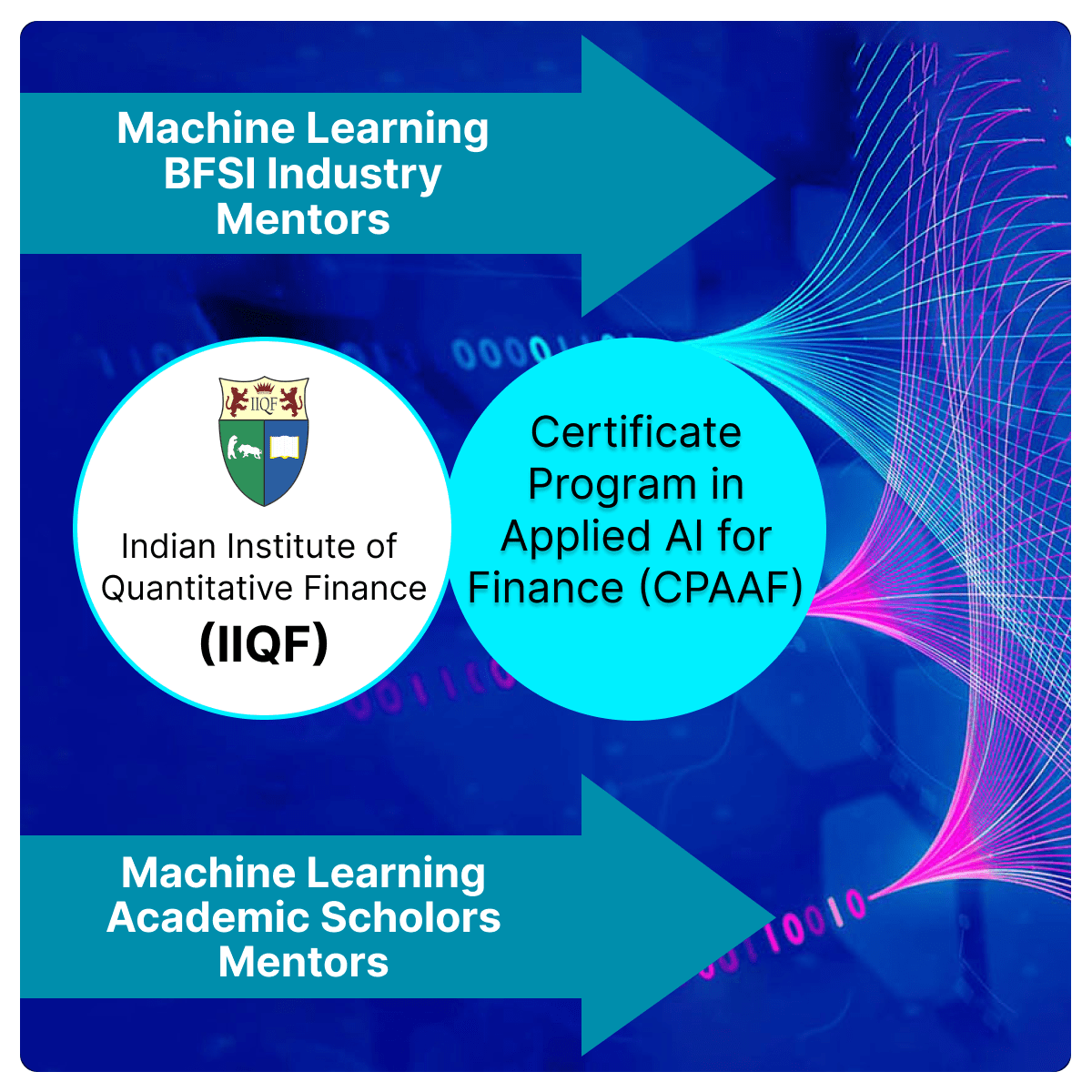
Rupal
Background
- B.Tech from IIT, Kanpur and Executive MBA from IIM Kozhikode.
- Currently working as Vice President, Fixed Income at one of the largest International Bank for their Corporate Investment Banking Division.
- Prior to this he was working as Assistant Vice President at Credit Suisse, Investment Banking Division.
- Regularly worked as an internal trainer in the organizations that he has worked in.
ML Expertise (Teaching ML Application for Financial Systems)
- Passionate about teaching, he has been conducting workshops and training programs on Machine Learning & Data Science.
- His areas of interest are fixed income pricing, financial analytics and statistical learning
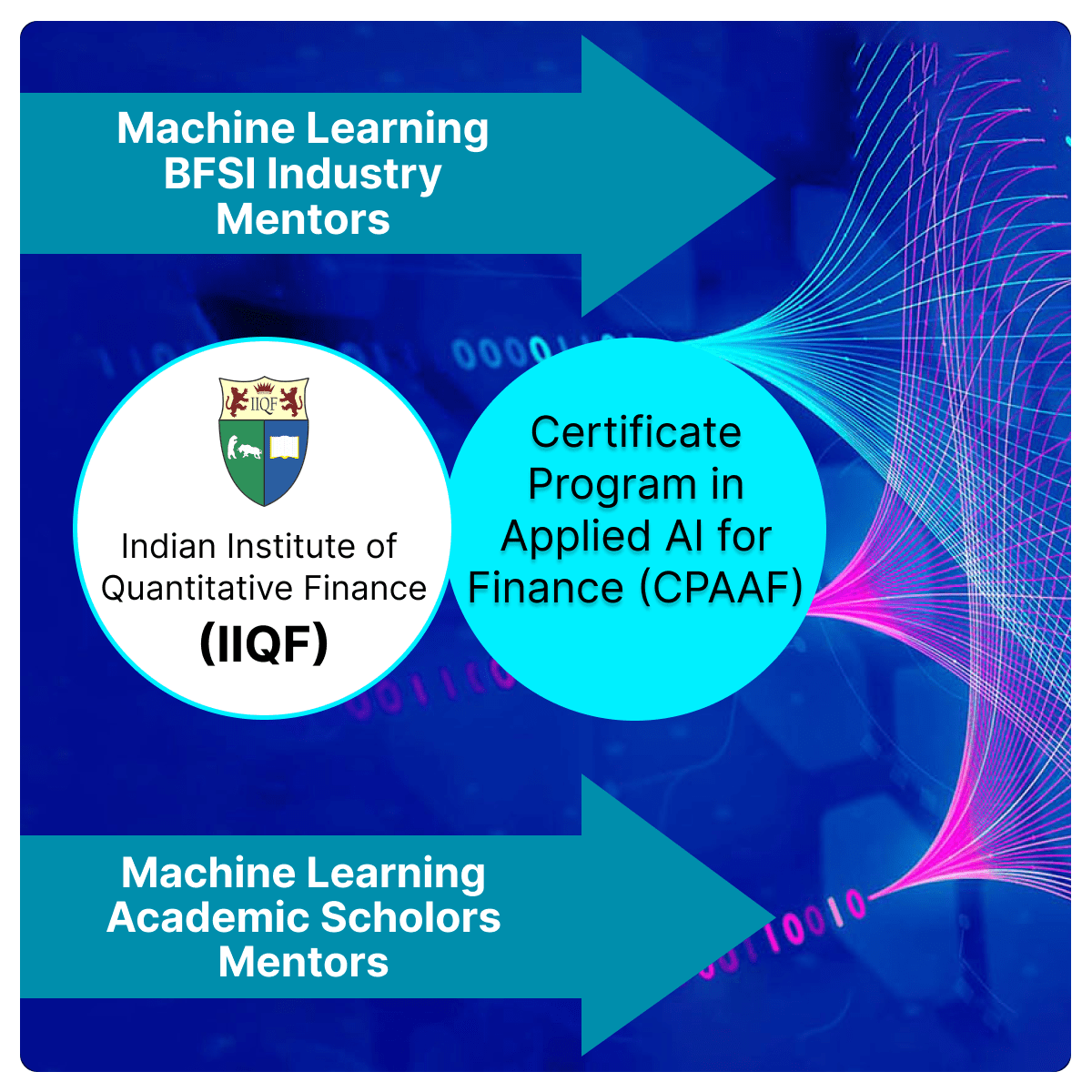
Dr. Hari
Background
- M.Sc. in Mathematics from B.H.U. Varanasi.
- Awarded four gold medals because of his outstanding performance in B.H.U.
- He has completed his Ph.D. in Mathematics from Indian Institute of Science, Bangalore.
- He has also worked with Deep Value (Algorithm trading firm).
- Currently involved with one of the world's leading analytics product company.
ML Expertise (Teaching ML Application for Algorithmic Trading)
- Passionate about teaching, he has been conducting workshops and training programs on Machine Learning for Trading.
- ML applications for Algorithmic Trading, High Frequency Trading & Quantitative Analytics
Admission Process in AI Risk Management Course
-
Send Your Application
-
Get on a call with a counsellor
-
Wait for Application Acceptance
-
Pay the fee & join the upcoming batch
Finance your Study
Educational Loans
We are very happy to help you progress to greater heights in your career in every way possible. Education loans available at 0% interest for full time Indian residents. Easy EMI plans available.
Student Aid
Encourages the full time students to enter this domain, benefits, if you are still pursuing formal education.
Get Answers
-
What are broader application use cases of Artificial Intelligence (AI) & Machine Learning (ML) in financial risk management?
AI & ML techniques are extensively employed in portfolio risk, credit risk, market risk & operational risk for prediction, forecasting, classification, segmentation, attribution, optimization problems in the broader sphere of risk modelling & analytics. -
What are the desired skill sets & core competencies to be a AI & ML expert in risk management domain?
AI & ML risk applications requires skill building in key learning areas like risk data mining & augmentation, risk data analytics & visualization, risk model building, AI & ML techniques, Explainable AI & Responsible AI, programming skills, AI & ML tech stack & toolset knowhow etc. -
How AI & ML models are more cutting edge than the conventional statistical models for risk management problem sets?
AI & ML models are far more capable of handling noisy data, modelling alternative datasets, building dynamic data-driven models, estimating non-linear relationships, solving high-dimensional problems & many more. -
What are specific application use cases of AI & ML models for risk management domain in the BFSI & Fintech financial sector?
AI & ML has several established & emerging use cases for risk management function in banks, financial institutions, funds, trading outfits, Fintect firms:- Risk Analytics -> Visualization & Reporting
- Portfolio Risk -> Portfolio Segmentation, Allocation, Risk Decomposition & Attribution, Scenario Analysis & Stress Testing
- Credit Risk -> Prediction of Probability of Default (PD), Loss Given Default (LGD), Exposure At Default EAD, IFRS9 ECL Provisions
- Market Risk -> Forecasting of Stock Price or Index, Forex Rates ,Volatility Loss or Value at Risk (VaR) and Market Sentiment Analytics
- Fraud Risk -> Anomaly Detection, Outlier Diagnostics, Fraud Analytics & Forensic Data Science
-
What is the future outlook of AI adoption for risk management in the BFSI & Fintech financial domain?
According to Allied Market Research, the market valuation for AI in banking stands at $160 billion in 2024 and is anticipated to reach $300 billion by 2030. -
What kind of domain expertise is catered by CPAIRM certification?
CPAIRM is a specialized certification covering AI & ML algorithms and their applications in risk management sub-domains like Portfolio Risk, Credit Risk, Market Risk, Fraud Risk, & others. This specialized application oriented course is designed to cover the essentials on risk modelling and AI & ML use cases for various risk types and tribes. -
Can you exemplify any AI & ML driven application use case for risk management?
Below briefly highlights an AI & ML use case for stock market prediction:- • Neural Network (NN) based models can be employed for more accurate market risk prediction tasks due to their basic property – nonlinearity.
- • Neural Network (NN) universal approximation property allows to examine a non-linear association between the conditional quantiles of a dependent variable & predictors.
- • NN-based forecasting model can be used in order to indicate possible downside or upside moves in the market, form appropriate market timing strategies and assess the evaluation of risk measures like volatility risk, VaR or loss risk etc.
-
What kind of AI specific career opportunities & avenues available in the broader risk management area within BFSI & Fintech space?
The BFSI, Fintech & Financial Product/services/consulting firms offer a variety of career avenues and roles like risk quants modeler, risk AI model validator, risk products AI researcher, risk or forensic data scientist, risk analytics expert, risk platforms engineer, risk AI systems developer, risk AI product owner & project manager. -
Are there any prerequisites required for CPGAIF certification?
CPAIRM requires prior AI & ML technique know-how and intermediate-level programming proficiency in Python.
Similar Courses
NEED HELP?
You can call us on +91-8976993621 or email us your contact details if you would like a call back
(This service is normally available between 9.00 AM and 9.00 PM all the day. At all other times, please submit an email request.)