- About CPMLF
- Fee & Course Calender
- Download Brochure
- Faculty
- Course Outline
- Course Placements
- Finance your Study
- Faqs
Certificate Program in Machine Learning for Finance (CPMLF)
Build your career as Machine Learning Specialist in Banking and Financial Services
- English
-
Indian Institute of Quantitative Finance
- Book a guidance call
Quick Facts
- Program Duration
- Program Schedule
- Program Timing
- Program Start Date
CPMLF Program - Machine Learning for Finance Course Highlights
- Extensive 200 hours of personalized live online instructor-led interactive lectures
- Faculty - Industry practitioners & academic researchers from BFSI machine learning, quants & risk domain
- Exhaustive primers & preliminaries - Basic familiarity (Scratch-up coverage in primers & preliminary modules)
- Online learning modules with curated content & case studies
- Focused implementation labs for end-to-end ML algorithm data, design development, deployment & debugging
- Capstone project & research article work for final evaluation
- BFSI ML domain filtered job search & interview prep-up
ML for Finance Course Framework
-
Covering the essentials to enable anyone/everyone to take ML for course
-
Personalized live lectures by MNC industry practitioners and ML academicians/researchers
-
Real world industry application exposure
About The Certificate Program in ML for Finance
Primers & Preparatory Modules
- Linear Algebra - Basic Familiarity (Scratch-up coverage in primers & preliminary modules)
- Statistics - Basic Familiarity (Scratch-up coverage in primers & preliminary modules)
- Probability - Basic Familiarity (Scratch-up coverage in primers & preliminary modules)
- Differential & Integral Calculus - Basic Familiarity (Scratch-up coverage in primers & preliminary modules)
- Programming & Coding - Basic Familiarity (Scratch-up coverage in primers & preliminary modules)
- Exploratory Data Analysis - Basic Familiarity (Scratch-up coverage in primers & preliminary modules)
- Financial Datasets - Basic-to-Medium Familiarity
Key Learning Outcomes
- Advanced Programming in analytically powerful languages like Python
- Data Science for data manipulations, transformations & visualizations
- Machine Learning (ML) Techniques – supervised, semi-supervised & unsupervised techniques
- Machine Learning (ML) Applications – Finance & Risk Management
- Machine Learning (ML) Applications – Designing Automated Decision Systems
- Machine Learning (ML) Applications – Algorithmic Trading & Investment Strategies
Industry Scope
- BFSI Data Scientist
- BFSI Data Engineer
- BFSI Machine Learning Developer
- BFSI Machine Learning Algo Designer
- BFSI Machine Learning Product Owner
- BFSI Machine Learning Researcher
- BFSI Computational Quant Researcher
- BFSI Quantum Computing Expert
- BFSI Data Science & Machine Learning Program/Project Manager
Batch | Start Date | Fee | Mode | Time |
---|
Why choose CPMLF Program?
View what our learners have to say...
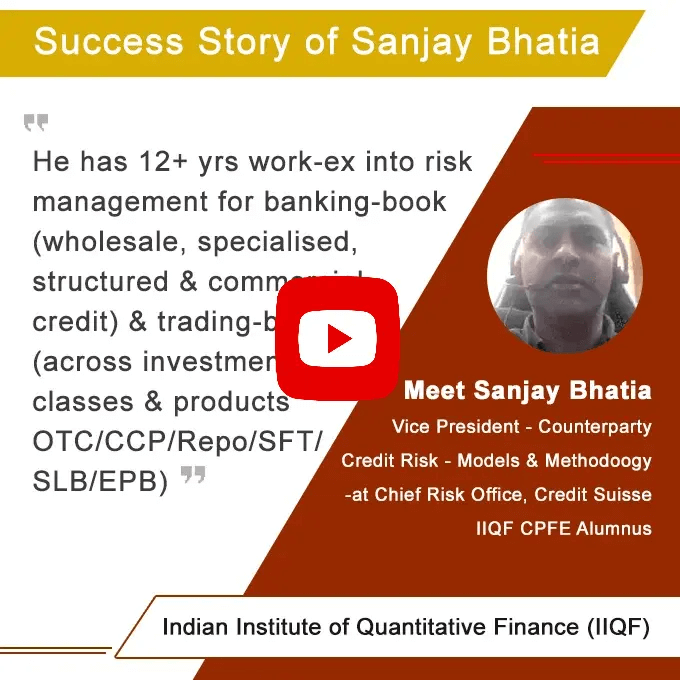
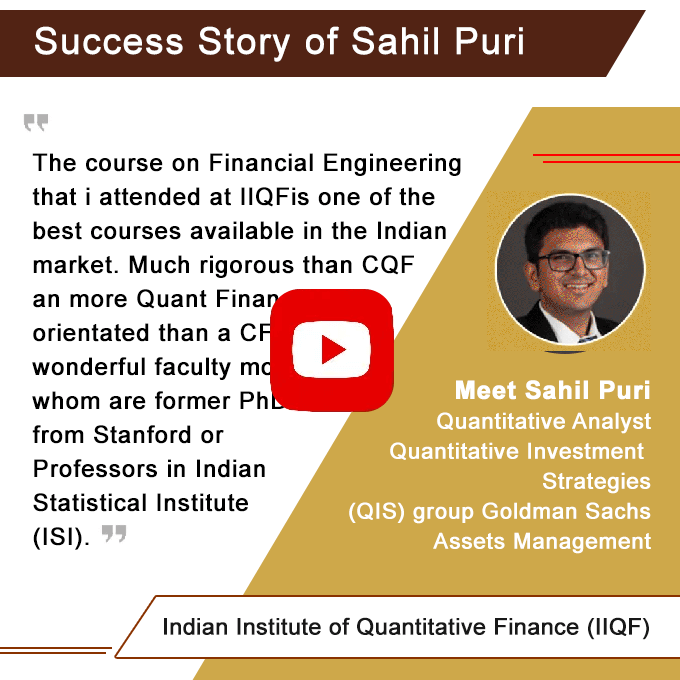
-
Learning Journey Step 110 Weeks
-
Learning Journey Step 210 Weeks
-
Learning Journey Step 310 Weeks
-
Application Journey Step4 Weeks
Machine Learning Preliminaries
- Preparatory ML Overview Primer
- Exhaustive ML Programming Primer
- Exhaustive Mathematical Primer
- Milestone Timeline - 6 Weeks
- Starting Point - CPMLF course starting time
- Support - Comprehensive Primers, Weekly Doubt Clearing Sessions, Toy Topical Sessions, Python Labs
-
- Starting Timeline
- Module0
- 4 Weeks
-
- CPMLF
- Course
- Inception
-
- End Timeline
- Module0
- 2 weeks
Machine Learning for Financial Applications
- ML Paradigms & Structural Framework
- Supervised Learning
- Semi-Supervised Learning
- Unsupervised Learning
- Deep Learning
- Online/Off-Line Learning
- Reinforcement Learning
- Financial Problem Settings & Use Cases
- ML Solution Design & Architecture
- ML Model Development Lifecycle
- Milestone Timeline – 4 Weeks
- Starting Point – Post Module 0
- Support – ML Problem Framing, ML Problem Solving, Financial Use Case Library, ML BFSI Applications, ML BFSI System Design
-
- Starting Timeline
- Module0
- 4 Weeks
-
- CPMLF
- Course
- Inception
-
- End Timeline
- Module0
- 2 weeks
-
- End Timeline
- Module1
- 4 weeks
-
Learning Journey Step 110 Weeks
-
Learning Journey Step 210 Weeks
-
Learning Journey Step 310 Weeks
-
Application Journey Step4 Weeks
ML Supervised Learning Algorithms
- ML Driven Regression
- ML Driven Time Series
- ML Driven Classification
- ML Driven Data Visualization
- ML Driven Data Science Insights & Story Telling
- Milestone Timeline – 6 Weeks
- Starting Point – Post Module 1
- Support – Weekly 8 hours of Live Lectures, Python Labs – Coding Practice, Supervised Learning Financial Use Case Studies
-
- CPMLF
- Course
- Inception
-
- CPMLF
- Learning
- Journey 1
-
- Learning
- Module2
- 6 weeks
ML Un-Supervised Learning Algorithms
- ML Driven Clustering
- ML Driven Association
- ML Driven Data Visualization
- ML Driven Data Science Insights & Story Telling
- Milestone Timeline – 4 Weeks
- Starting Point – Post Module II
- Support – Weekly 8 hours of Live Lectures, Python Labs – Coding Practice, Un-Supervised Learning Financial Use Case Studies
-
- CPMLF
- Course
- Inception
-
- CPMLF
- Learning
- Journey 1
-
- Learning
- Module2
- 6 weeks
-
- Learning
- Module3
- 4 weeks
-
Learning Journey Step 110 Weeks
-
Learning Journey Step 210 Weeks
-
Learning Journey Step 310 Weeks
-
Application Journey Step4 Weeks
Machine Learning Speech & Language Processing
- Unstructured Data Sets & Transformations
- ML Driven Textual & Speech Processing
- ML Driven Document Classification
- ML Driven Image Classification
- ML Driven Chat-bots
- Milestone Timeline – 6 Weeks
- Starting Point – Post Module III
- Support – Weekly 8 hours of Live Lectures, Python Labs – Coding Practice, Language & Image Processing Financial Use Case Studies
-
- CPMLF
- Course
- Inception
-
- Learning
- Journey 1
- 10 Weeks
-
- Learning
- Journey 2
- 10 Weeks
-
- Learning
- Module4
- 6 weeks
Machine Learning Evaluation & Explainability
- ML Performance Evaluation Metrics
- ML Quantitative Validation Tests
- ML Driven Model Explainability
- ML Driven Tuning & Optimization
- ML Results Showcase & Storytelling
- Milestone Timeline – 4 Weeks
- Starting Point – Post Module IV
- Support – Weekly 8 hours of Live Lectures, Python Labs – Coding Practice, ML Model Regulatory Prerequisites, Transparency & Explainibility for Financial Use Cases
-
- Learning
- Journey 1
- 10 Weeks
-
- Learning
- Journey 2
- 10 Weeks
-
- Learning
- Module4
- 6 weeks
-
- Learning
- Module5
- 4 weeks
-
Learning Journey Step 110 Weeks
-
Learning Journey Step 210 Weeks
-
Learning Journey Step 310 Weeks
-
Application Journey Step4 Weeks
Machine Learning Prototyping
- ML Front-To-Back Tech Stack
- ML Design & Architecture
- ML Prototyping
- ML Deployment
- ML Productionization
- Milestone Timeline – 2 Weeks
- Starting Point – Post Module V
- Support – Weekly 8 hours of Live Lectures, Python Labs – Front-To-Back ML Model Development & Deployment
-
- Learning
- Journey 1
- 10 Weeks
-
- Learning
- Journey 2
- 10 Weeks
-
- Learning
- Journey3
- 4 weeks
-
- Learning
- Module6
- 2 weeks
Machine Learning Capstone Project
- Quantitative Finance
- Derivative Pricing
- Risk Modelling & Management
- Portfolio Management
- Algorithmic Trading
- Financial Time Series Forecasting
- Milestone Timeline – 2 Weeks
- Starting Point – Post Module VI
- Support – Mentoring Support, Thesis Supervisor Support, Feedback Supports
-
- Learning
- Journey 1
- 10 Weeks
-
- Learning
- Journey 2
- 10 Weeks
-
- Learning
- Journey3
- 4 weeks
-
- Learning
- Journey4
- 6 weeks
Faculty
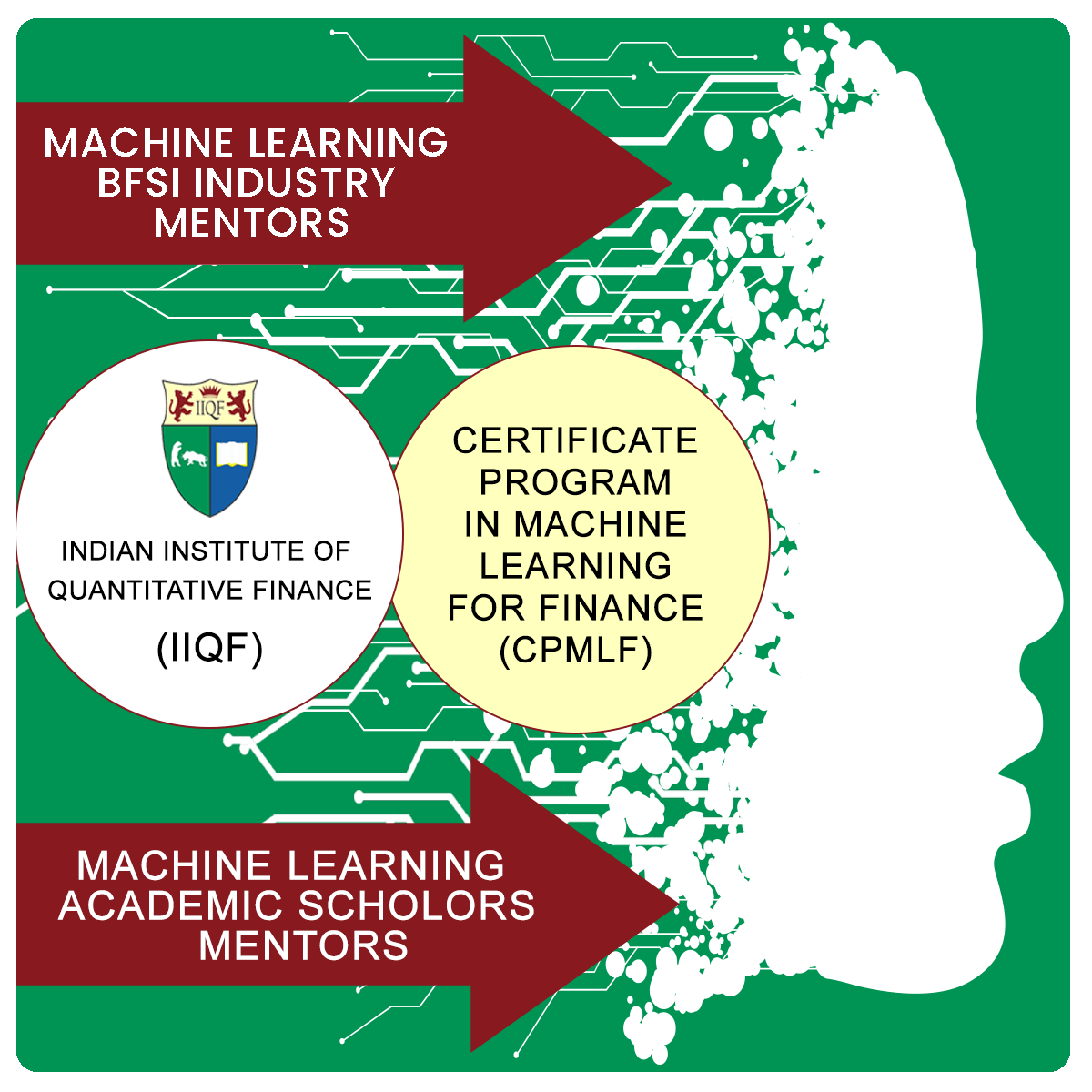
SANJAY BHATIA
Background
- Director in UBS - Risk Modelling & Analytics, Model Risk Management & Control, Chief Risk Office (CRO) Function
- MBA-Finance & MSc in Machine Learning & Artificial Intelligence from Liverpool John Moores University (LJMU)
- Post-Graduate Diploma in Machine Learning & Artificial Intelligence from IIIT-Bangalore
- Domain SME on Credit Risk , Derivatives Counterparty Credit Risk, Derivative Pricing, Stochastic Modelling, Stress Testing
ML Expertise (Teaching ML for Quantitative Finance & Risk Management)
- Financial Prediction (Regression & Classification ) - Lasso/Ridge Regression, CART Decision Trees, Ensemble Learning (Bagging & Boosting) & Support Vector Machines (SVM)
- Financial Time Series Forecasting - (Recurrent) Neural Networks, RNN-LSTM, RNN-GRU, Hybrid-RNN-LSTM-GRU
- Financial Instrument Pricing - Non-Linear & High Dimensional Derivative Pricing using Neural Networks
- ML Model Optimization – Hyperparameters Tuning K-Fold Cross-Validation, Stochastic Gradient Descent, Convergence etc.
- Regulatory & Industry ML Adoption, Challenges & Use Cases – Model Explainibility, Performance Evaluation & Testing
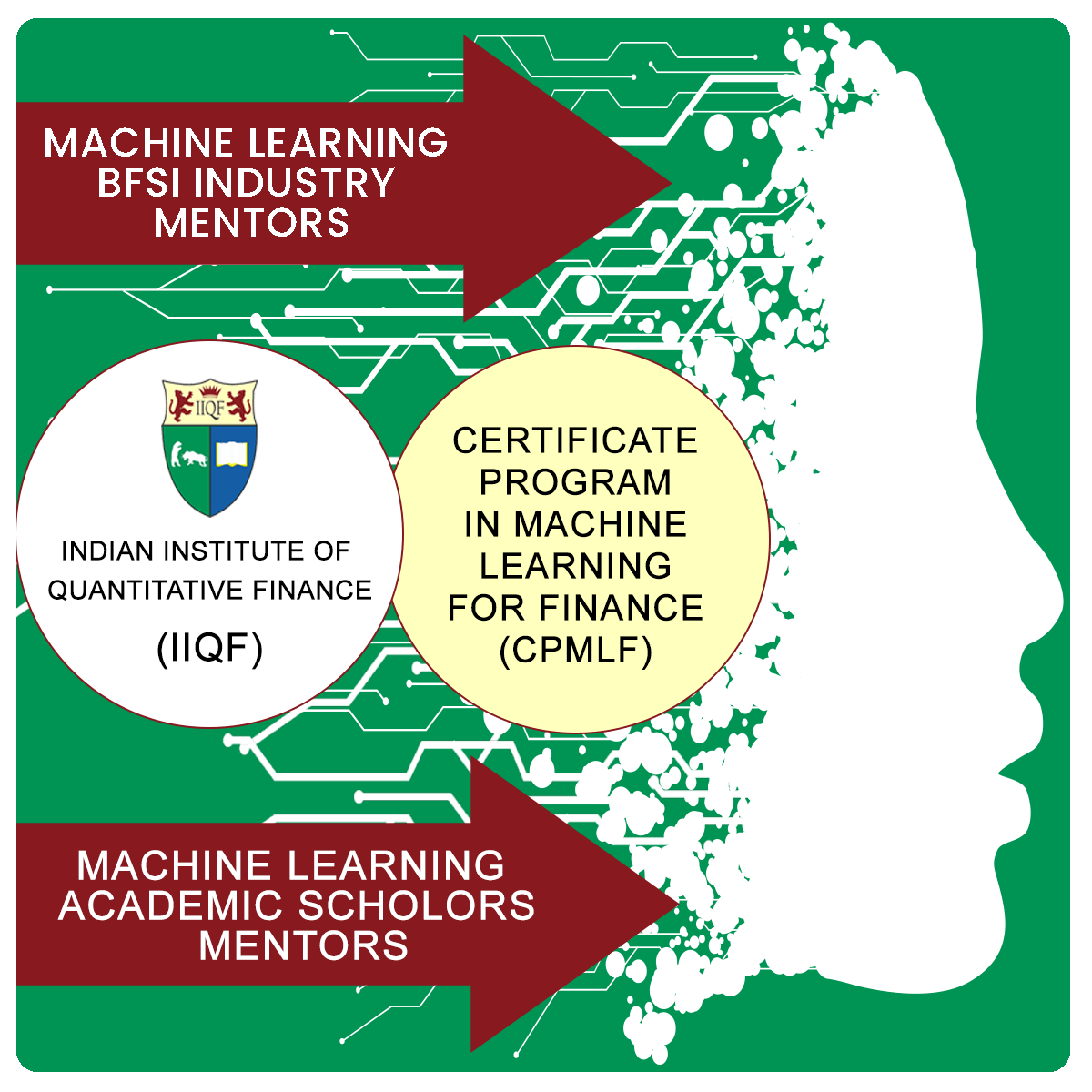
Prof Rituparna Sen
Background
- - Associate Professor at the Applied Statistics Division, Indian Statistical Institute, Bangalore.
- - She worked as Assistant Professor at the University of California at Davis from 2004–2011
- - She has also taught courses in Chennai Mathematical Institute and Madras School of Economics.
- - An elected member of the International Statistical Institute
- - A council member of the International Society for Business and Industrial Statistics.
- - She has been awarded the Young Statistical Scientist Award by the International Indian Statistical Association, the Best - Student Paper Award by the American Statistical Association and the Women in Mathematical Sciences award by Technical University of Munich, Germany.
ML Expertise (Teaching ML Statistics for Computational Finance)
- - Authored A Book on Computational Finance with R
- - Authored 30+ Research Papers & Articles
- - Editor: Journal of Applied Stochastic Models in Business and Industry
- - Member of CAIML (Center for Artificial Intelligence and Machine Learning) at ISI
- - Associate Editor of several other journals
- - Guided several masters students on theses in the ML area
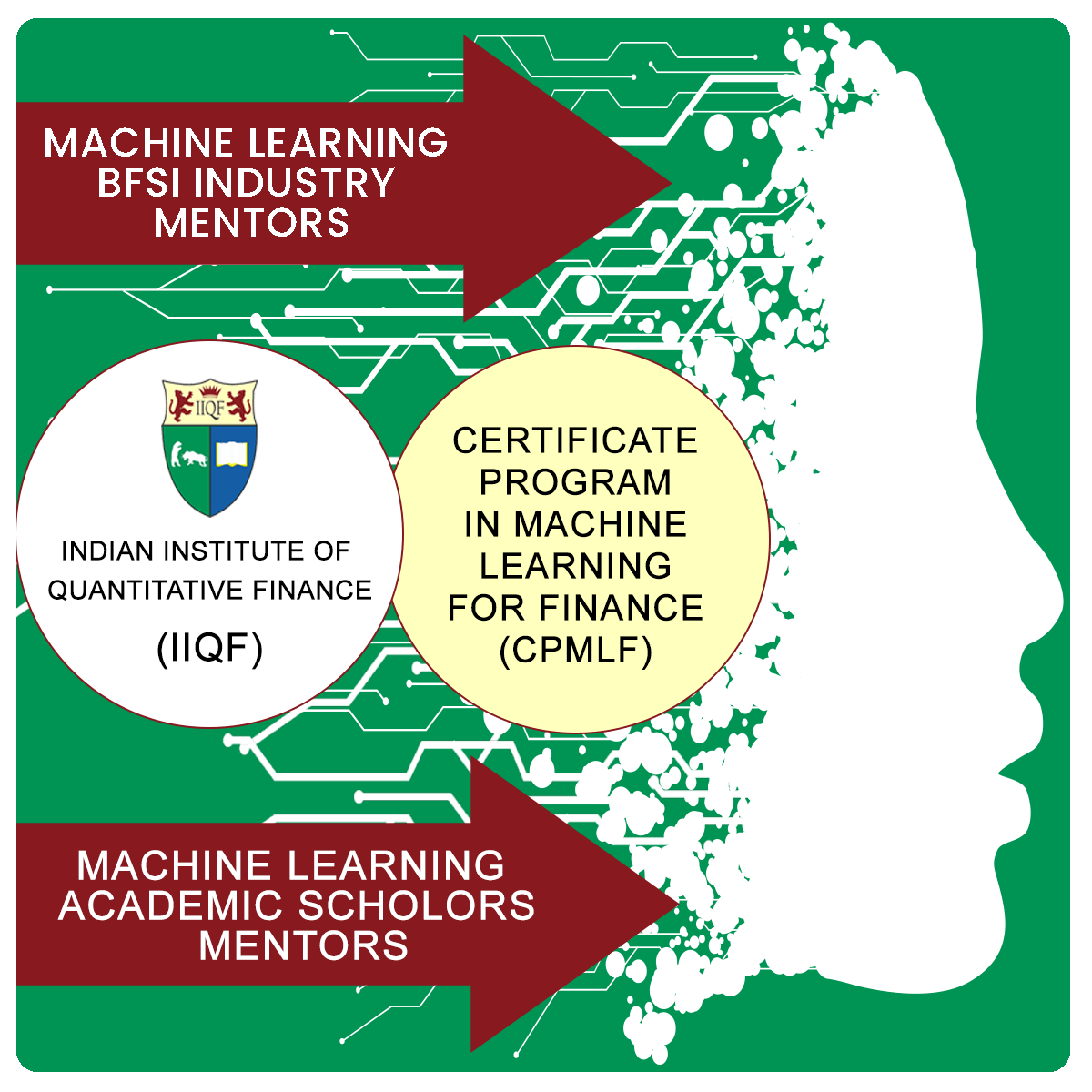
Dr. Arindam Chaudhuri
Background
- Worked as post doctoral fellow with Department of Computer Science, University of Copenhagen and Department of Computer Science, Technical University of Berlin.
- Worked as researcher with Siemens Research Labs Amsterdam and Samsung Research Labs at New Delhi & Bangalore.
- His current research interests include business analytics, artificial intelligence, machine learning, deep learning.
- He has published 4 research monographs and 60 articles in international journals and conference proceedings.
- He has served as reviewer for several international journals and conferences.
ML Expertise (Teaching ML Statistics for Computational Finance)
- Implemented Machine Learning Methods - Support Vector Machines, Artificial Neural Networks, Deep Learning Networks, Clustering, Genetic Algorithms and Evolutionary Computing with basic mathematical foundations of probability theory, fuzzy sets, rough sets, possibility theory and a variation of these for solution of various business problems.
- Integrated various artificial intelligence methods to form different soft computing frameworks such as neuro-fuzzy, fuzzy-genetic, neuro-genetic and rough-neuro-fuzzy-genetic.
- Successfully applied these methods for different categories of industrial problems such as decision theory, time series forecasting and prediction, image compression, sentiment analysis, recommendation systems, social networks analytics in order to achieve better results
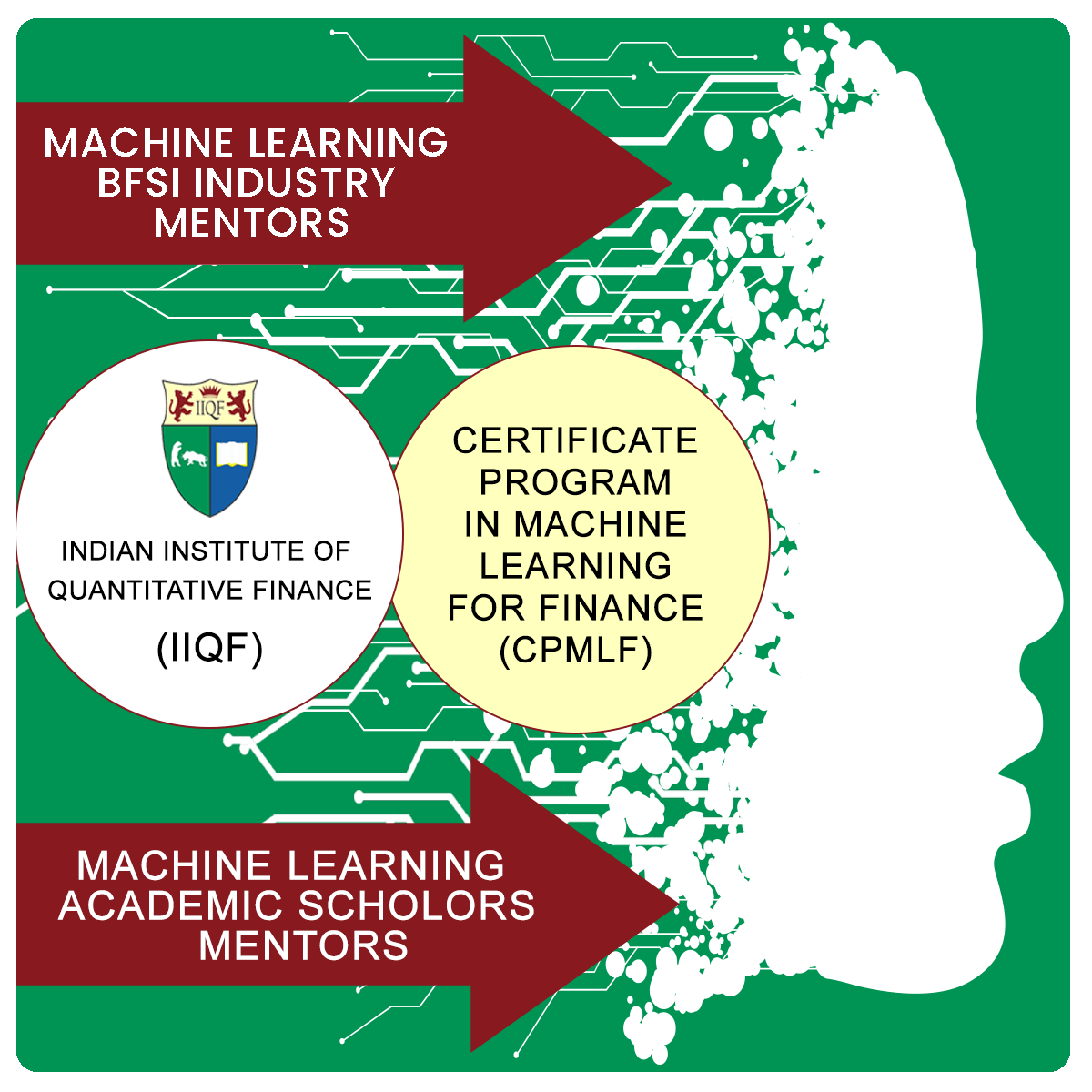
Ritesh Chandra
Background
- - B. Tech. (IIT-Kanpur), PGDM (IIM-Calcutta), CFA
- - 15 years banking experience in risk management with domestic and MNC banks
- - Member, Board of Studies in the area of Finance at IMT-CDL, Ghaziabad
ML Expertise
- - Passionate about teaching. Has been conducting workshops / training programmes for the last 8 yrs in areas of Quantitative Finance, Financial Management, Risk Management and Machine Learning
- - Has contributed to a book on Applications of Blockchains in Financial Services industry
- - Has worked as visiting faculty with several institutions.
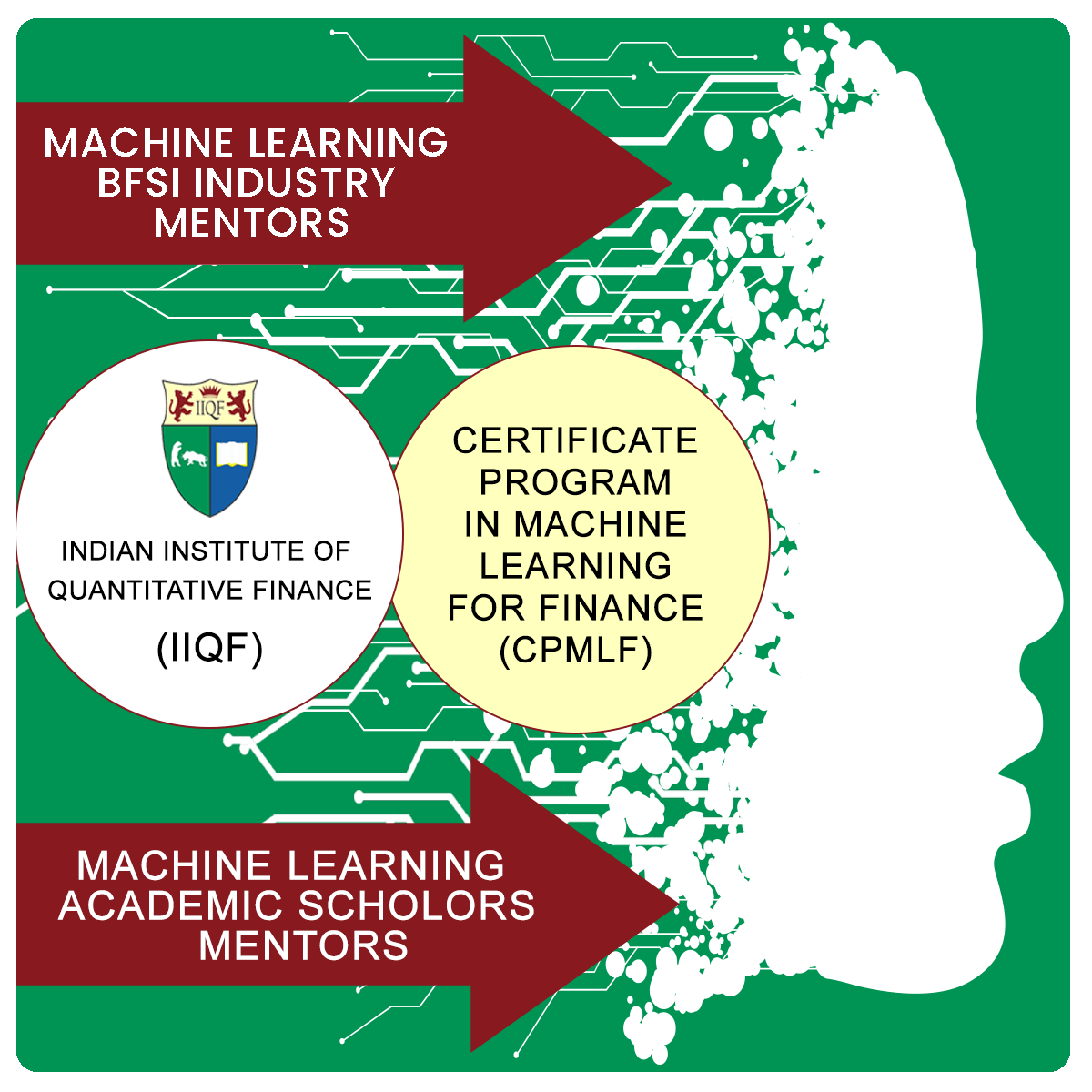
Rupal
Background
- B.Tech from IIT, Kanpur and Executive MBA from IIM Kozhikode.
- Currently working as Vice President, Fixed Income at one of the largest International Bank for their Corporate Investment Banking Division.
- Prior to this he was working as Assistant Vice President at Credit Suisse, Investment Banking Division.
- Regularly worked as an internal trainer in the organizations that he has worked in.
ML Expertise (Teaching ML Application for Financial Systems)
- Passionate about teaching, he has been conducting workshops and training programs on Machine Learning & Data Science.
- His areas of interest are fixed income pricing, financial analytics and statistical learning
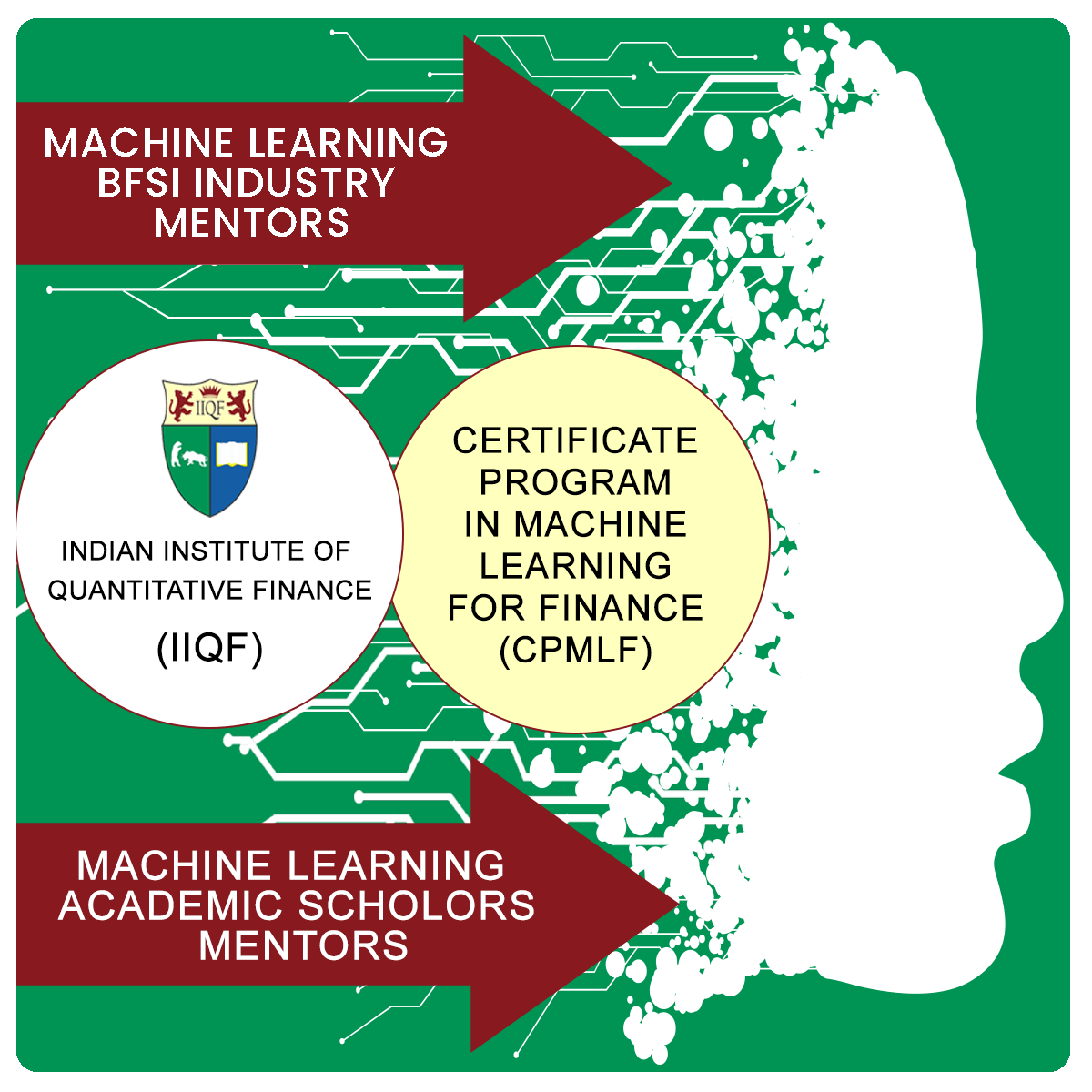
Dr. Hari
Background
- M.Sc. in Mathematics from B.H.U. Varanasi.
- Awarded four gold medals because of his outstanding performance in B.H.U.
- He has completed his Ph.D. in Mathematics from Indian Institute of Science, Bangalore.
- He has also worked with Deep Value (Algorithm trading firm).
- Currently involved with one of the world's leading analytics product company.
ML Expertise (Teaching ML Application for Algorithmic Trading)
- Passionate about teaching, he has been conducting workshops and training programs on Machine Learning for Trading.
- ML applications for Algorithmic Trading, High Frequency Trading & Quantitative Analytics
Financial Engineering
Modern Investment Finance is hugely dependent on the implementations of the theories and techniques of financial engineering. Financial Engineering, or Quantitative Finance as it is alternately known, is a multidisciplinary field involving the application of theories from financial economics, physics, mathematics, probability, statistics, operations research and econometrics using the methods and tools of engineering and the practice of computer programming to solve the problems of Investment Finance.
Generally the language of choice for Quant implementations traditionally has been C++ along with tools like Matlab, Mathematica, Stata, etc. However, of late the Python language has become more popular.
Financial Engineering has emerged as a very prospective career prospect for people with a strong mathematical background like those coming from engineering, mathematics, statistics, physics or econometrics background. The best of the global financial institutions like Investment Banks, Hedge Funds, etc. hire people having strong quantitative skills for “Quant” jobs. This is also a very rewarding and exciting career option for such people as there is ample scope for applying their numerical and creative skills to design new things, be it devising new investment strategies or be it structuring new financial instruments or be it finding methods to value them. They are continuously competing with their peers and some of the best minds in the market and have to out-perform them to generate superior returns, which is intellectually a very challenging work, and this makes it all the more thrilling.
Brief Financial Engineering Courses Outline
-
Preparatory PrimersCeritificate Program in Machine Learning and Data Science for Quant Finance
- ML Programming Preliminaries
- ML Mathematical Preliminaries
- ML Probability & Statistical Preliminaries
-
Module ICeritificate Program in Machine Learning and Data Science for Quant Finance
- Machine Learning Overview, Framework & Finance Applications
-
Module IICeritificate Program in Machine Learning and Data Science for Quant Finance
- Machine Learning Supervised Learning Algorithms
-
Module IIICeritificate Program in Machine Learning and Data Science for Quant Finance
- Machine Learning Unsupervised Learning Algorithms
-
Module IVCeritificate Program in Machine Learning and Data Science for Quant Finance
- Machine Learning Speech and Language Processing
-
Module VCeritificate Program in Machine Learning and Data Science for Quant Finance
- Machine Learning Model Evaluation Metrics and Model Validation
Financial Engineering Career Opportunities
Modern Investment Management has become very much mathematical and statistical in nature, it is now much more of science than arts, specially where investments in complex financial instruments and complex trading/investment strategies are concerned. That is the reason that high-end investment firms that invest in derivatives are opting for people who have strong quantitative skills for structuring or valuation of complex financial instruments and for devicing superior investment strategies.
This has opened up very exciting and rewarding career opportunities in the field of Quantitative Investment Management for candidates who come with academic background in engineering, mathematics, and other numerical specializations. Needless to say, that apart from the stimulating intectual challenges that careers in this field offer to the mathematically talented individuals, the compensations are quite handsome indeed.
The course prepares candidates for careers as quantitative investment managers or quantitative analysts with financial institutions like investment banks, hedge funds, private equity firms, large broking houses, investment research and analytics firms, etc.
Candidates having a strong numerical background have a very bright chance of making a very rewarding career in this field with the largest of investment banks and other financial institutions. Salaries of quantitative analysts vary depending on their experience and background. In India presently, salaries for this profile may range from 8 Lacs p.a. for freshers to 30 Lacs p.a. for candidates with a few years of experience.
CPMLF Course Placement
Students completing Certificate Program in Machine Learning Finance online courses will get placement assistance subject to the fulfilment of applicable conditions.
Admission Process in CMPLF Program
-
Send Your Application
-
Get on a call with a counsellor
-
Wait for Application Acceptance
-
Pay the fee & join the upcoming batch
Finance your Study
Educational Loans
We are very happy to help you progress to greater heights in your career in every way possible. Education loans available at 0% interest for full time Indian residents. Easy EMI plans available.
Student Aid
Encourages the full time students to enter this domain, benefits, if you are still pursuing formal education.
Get Answers
-
To whom this special program in Machine Learning (ML) is applicable ?
This exhaustive ML program broadly caters to anyone & everyone looking for career avenues in emerging field of Machine Learning & Data Science irrespective of industry/sector yet we’ve designed the application part to specifically cover implementation use cases in below sub-fields:
- Accounting & Finance
- Risk Management
- Portfolio Analytics
- Trading & Investment Analysis
- Regulatory & Internal Compliance
- Computational Finance & Financial Engineering -
What potential career avenues are available in the industry for the aspirants of this ML program?
This ML program caters to building practitioner-level skills to broadly cater to any & all ML & Data Science driven role families with a few real examples from the job market given below :
- Data Scientist for Financial/Accounting Decision Systems
- Machine Learning Model Designer for Financial Forecasting & Predictive Models
- Forensic Audit Data Scientist
- Fraud Analytics Machine Learning Expert
- Risk/Finance/Compliance Machine Learning Data Engineer
- Machine Learning Modeler for Quantitative Financial Models
- Machine Learning Driven Algorithmic Trader
- Machine Learning Model Validation Expert -
What skills one should possess to pursue Machine Learning & Data Science as a futuristic career path?
- Machine Learning Technical Know-How - E.g. Supervised/Unsupervised/Reinforcement/Deep-Learning Techniques & Methods
- Programming & Coding Skills - E.g. High Level & Powerful Languages Python, Julia, specific libraries/modules in SAS/R etc.
- Mathematical Skills - E.g. Linear Algebra, Matrix & Vector Operations, Multivariate Calculus, Optimization Problems
- Probability Theory & Statistical Skills - E.g. Probability Rules, Expectation, Conditional and Joint Distributions, Standard Distributions (Bernoulli, Binomial, Multinomial, Uniform and Gaussian) -
What is the ML for Finance course structure & design?
ML for Finance Course Design FrameworkCovering the essentials to enable anyone/everyone to take this course- ML Programming Preliminaries
- ML Mathematical Preliminaries
- ML Probability & Statistical PreliminariesPersonalized live lectures from ML academicians & SME industry practitioners- ML Methodology
- ML Finance Use Case ImplementationFull blown pragmatic learning exposure- ML Python Labs with full time technical assistance
- ML Finance Use Case Implementation
- Capstone Project
- Hackathon Style Contests -
What kind of machine learning application use cases covered by CPMLF?
- Financial Time Series (Price, Volatility, VaR, ES etc.) Forecasting using Deep Learning Neural Networks (NN) algorithms
- Credit and counterparty credit risk modelling (PD, LGD, EAD, XVA, Margining etc.) using Ensemble Learning ML techniques
- High Dimensionality Reduction using Principal Component Analysis (PCA)/Autoencoder
- Derivative Pricing using Deep Learning Neural Networks (NN) algorithms
- Investment Portfolio Construction & Optimization using Reinforcement Learning
- Sentiment analysis on unstructured financial data using NLP algorithms
- Many others…. -
How machine learning (ML) models disrupt the world of quant finance?
- ML algorithms provides data-driven analytics versus assumption or theory based classical statistical models
- ML algorithms are more proficient to handle non linearities, multicollinearities, curvature risk and tail risk
- ML algorithms are more faster & efficient than statistical models
- ML algorithms allows for more dynamic estimations, parameterizations and calibrations
- ML algorithms can be made more consistent with front-office models leading to front-to-back integration
- ML algorithms facilitates real time portfolio risk management, asset pricing and valuation
- Many more….. -
What machine learning allied competencies are built by CPMLF course?
- Big Data & Processing Tech Stack
- Data Engineering, Exploration and Exploitation
- Data Synthetization & Simulations
- Data Insights, Visualization & Story Telling
- ML Devops - Design, Development, Debugging and Deployment
- ML model fine-tuning and optimization
- ML Research Expeditions
- Many more….. -
How CPMLF course is different from other such AIML course offerings?
- Upstarter primers & preliminaries for scratch-up skill building - ML Stats, Probability, Math & Programming
- Faculty includes - BFSI industry top senior ML practitioners & academia researchers
- ML pragmatic hands-on learning approach – focused problem solving, BFSI case studies, ML financial use case library etc.
- Exhaustive course coverage - ML Supervised, Semi-Supervised & Unsupervised Techniques
- 250+ hours of live classroom lectures
- Python prototyping labs & implementation workshops
- ML front-to-back tech stack & deployment
- Financial, Risk & Quants specific applications
- ML capstone projects, research topics & white paper writing
- Much more in-store…. -
What additional support is expected to be rendered by IIQF®️ for CPMLF students?
- Capstone & research project mentoring
- BFSI industry ML core competencies awareness
- Placement opportunities for machine learning, data science, quantitative modelling & analytics role families
- Career guidance & coaching – skill evaluation, CV building, opportunity prospecting etc.
- Mock-up interview preparations & grooming
- Much more in-store….. -
What my CPMLF learning journey will look like if I don’t posses any of the basis skills & awareness on Machine Learning careers in finance?
- You will get lot of preparatory primers & preliminaries to get you the basic essential skills for machine learning
- You will get personal mentoring to get you doubts clarified & get you additional resources to build in basics right
- You will get the holistic know-how of programming & regular python labs practice sessions on coding problems
- You will get tailor made use cases & dataset aligned to your area of interest for prototyping
- You will get career guidance & counselling sessions to plan your aspirational career goal seeking
- You will get the right suitable job opportunities & targeted advise on how to shape up yourself as a prospective candidate
- Much more in-store….
NEED HELP?
You can call us on +91-8976993621 or email us your contact details if you would like a call back
(This service is normally available between 9.00 AM and 9.00 PM all the day. At all other times, please submit an email request.)