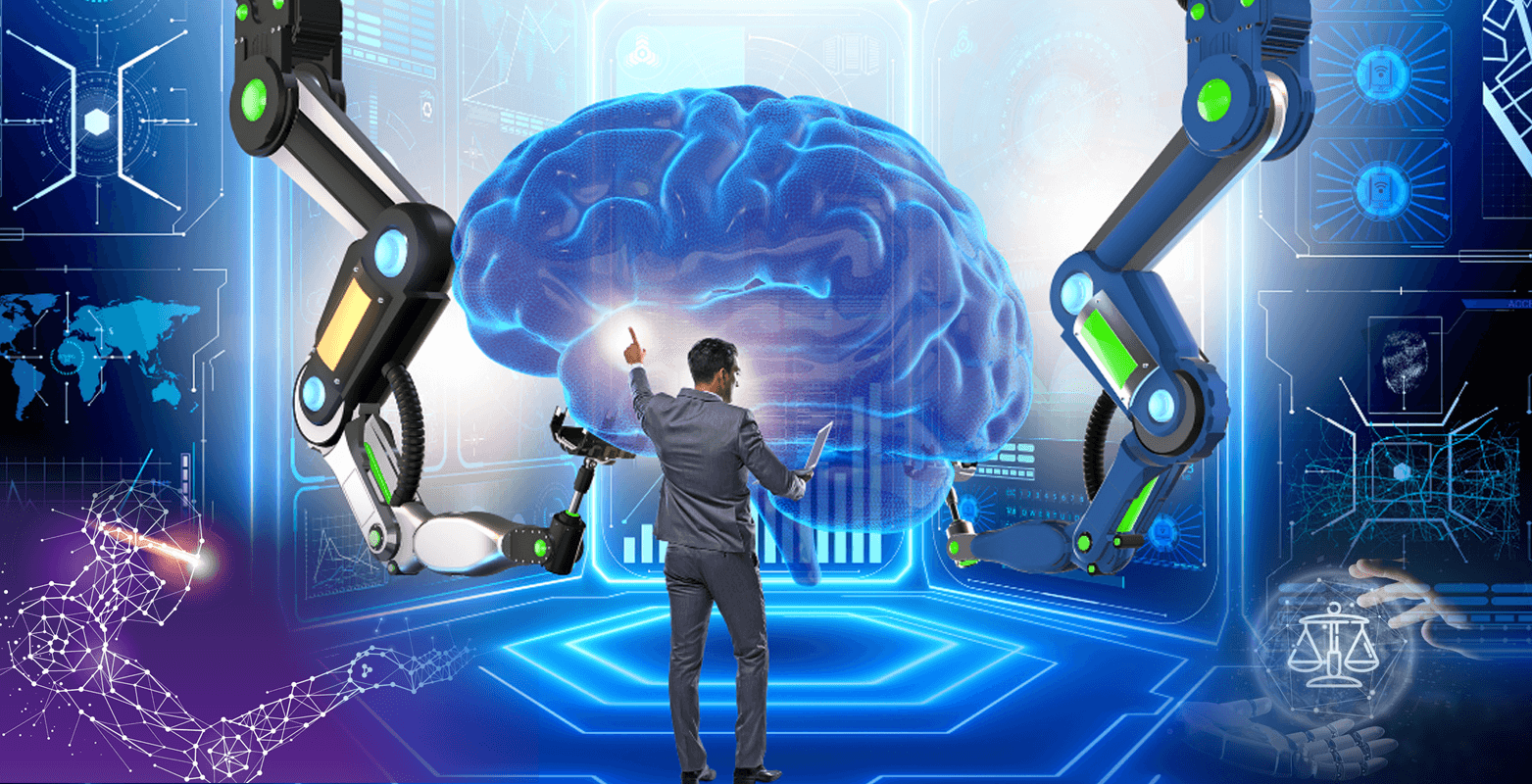
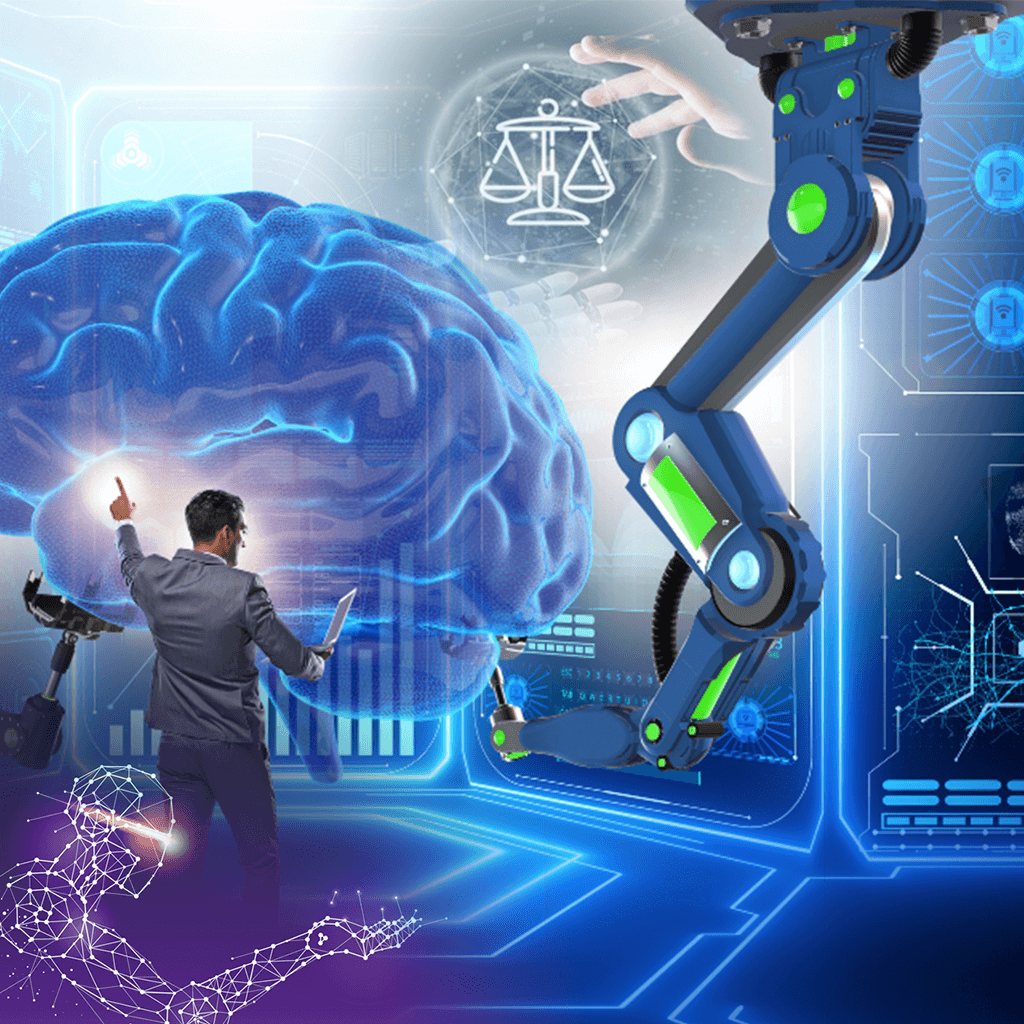
Profits vs. Principles: Ethical Hurdles of Machine Learning in Quantitative Finance
In the exhilarating world of quantitative finance, where algorithms and data reign supreme, integrating machine learning has introduced a new paradigm. The promise is clear: improved decision-making, increased profitability, and a more efficient financial system. Yet, as we tread this path paved with algorithms, data, and dreams of wealth, we must pause and consider the ethical implications.
Machine learning in quantitative finance has brought not only profits but a host of principles and dilemmas. The power to analyse vast datasets, optimise trading strategies, and manage risks has raised ethical questions that can't be ignored. In this blog, we will explore the ethical challenges associated with machine learning in quantitative finance and provide a structured approach to navigate these treacherous waters.
The Ethical Dilemmas
Ethical dilemmas that stem from its data-driven approach and automation prowess mar machine learning's journey in quantitative finance.
- 1. Data Privacy and Security:
As machine learning algorithms feed on data, the use of sensitive financial information raises concerns about data privacy and security. Financial institutions must tread carefully, ensuring they comply with data protection regulations and safeguard against data breaches and cyberattacks.
- 2. Algorithmic Bias:
The algorithms' ability to uncover hidden patterns in historical data is both a boon and a bane. If historical data contains biases, and such biases have quite often been found in several data instances like for example in credit data, the machine learning model may inadvertently perpetuate them, resulting in discriminatory outcomes. Quantitative professionals must address and mitigate algorithmic bias to ensure fairness.
- 3. Market Manipulation:
The automation and speed of machine learning-driven trading raise the spectre of market manipulation. The swiftness of algorithmic trading can distort market dynamics. To be ethical, professionals must adhere to market regulations and maintain market integrity.
- 4. Transparency and Explainability:
Understanding how machine learning models make decisions can be difficult because of their black box nature. This lack of transparency can hinder accountability and raise concerns about fairness. Professionals must strive to ensure transparency and explainability in their algorithms.
- 5. Impact on Employment:
The automation of financial tasks through machine learning can lead to job displacement in the industry. Professionals must consider the social and ethical implications of this shift and explore ways to support affected individuals.
Tackling the Ethical Quandaries
The ethical challenges posed by machine learning in quantitative finance are complex, but professionals can navigate them with strategic approaches.
- 1. Comprehensive Training:
The first step to addressing ethical challenges is education. Pursuing specialised training programs, such as those offered by the Indian Institute of Quantitative Finance (IIQF), equips professionals with a deeper understanding of the ethical implications of machine learning in finance. These programs provide insights into best practices and regulatory compliance.
- 2. Ethical Frameworks:
Ethical frameworks are essential. Professionals must develop and adhere to ethical guidelines that govern the use of machine learning in financial decision-making. These guidelines should address data privacy, transparency, fairness, and other ethical concerns.
- 3. Data Scrutiny:
Professionals should scrutinise data sources and identify potential biases or ethical concerns in the data used to train machine learning models. Rigorous data cleansing and augmentation strategies can help minimise bias in data.
- 4. Algorithmic Fairness:
Implementing fairness-aware machine learning techniques can identify and mitigate bias in algorithms. Ethical quantitative professionals must ensure that their models do not discriminate against any group based on sensitive attributes.
- 5. Regulatory Compliance:
Staying current with financial regulations is crucial. Compliance is essential for maintaining ethical practices and ensuring the legal and responsible use of technology. Professionals must understand and adhere to regulations related to machine learning in finance.
The IIQF Advantage
To navigate the ethical hurdles of machine learning in quantitative finance, professionals can turn to the Indian Institute of Quantitative Finance (IIQF) for specialised education.
IIQF's Approach to Ethics:
IIQF's programs go beyond algorithms and numbers. They emphasise the importance of principles and ethics in financial decision-making. Courses offered by IIQF provide knowledge on the ethical considerations of machine learning in quantitative finance, regulatory compliance, and best practices.
Networking Opportunities:
Enrolling in an IIQF course not only provides knowledge but also offers access to a network of professionals committed to ethical quantitative finance. The opportunity to collaborate with like-minded individuals in the industry can be invaluable for addressing ethical challenges.
Hands-On Experience:
IIQF's curriculum provides hands-on experience in navigating the ethical challenges of machine learning in finance. This practical approach ensures that professionals are not only proficient in machine learning but also ethical leaders in the field.
Conclusion: An Ethical Roadmap in Quantitative Finance
As the world of quantitative finance continues to evolve, integrating machine learning becomes increasingly critical. The potential for profit and progress is immense, but it comes with ethical challenges that require conscientious addressing.
Professionals in quantitative finance must embrace ethical frameworks, comprehensive training, and ongoing learning to navigate the financial landscape responsibly. With the guidance and knowledge provided by institutions like IIQF, they can harness the power of machine learning while upholding principles that prioritise fairness, transparency, and responsibility.
In the end, the choice between profits and principles doesn't have to be a dilemma. It can be a harmonious partnership in the world of quantitative finance, guided by ethics and expertise.