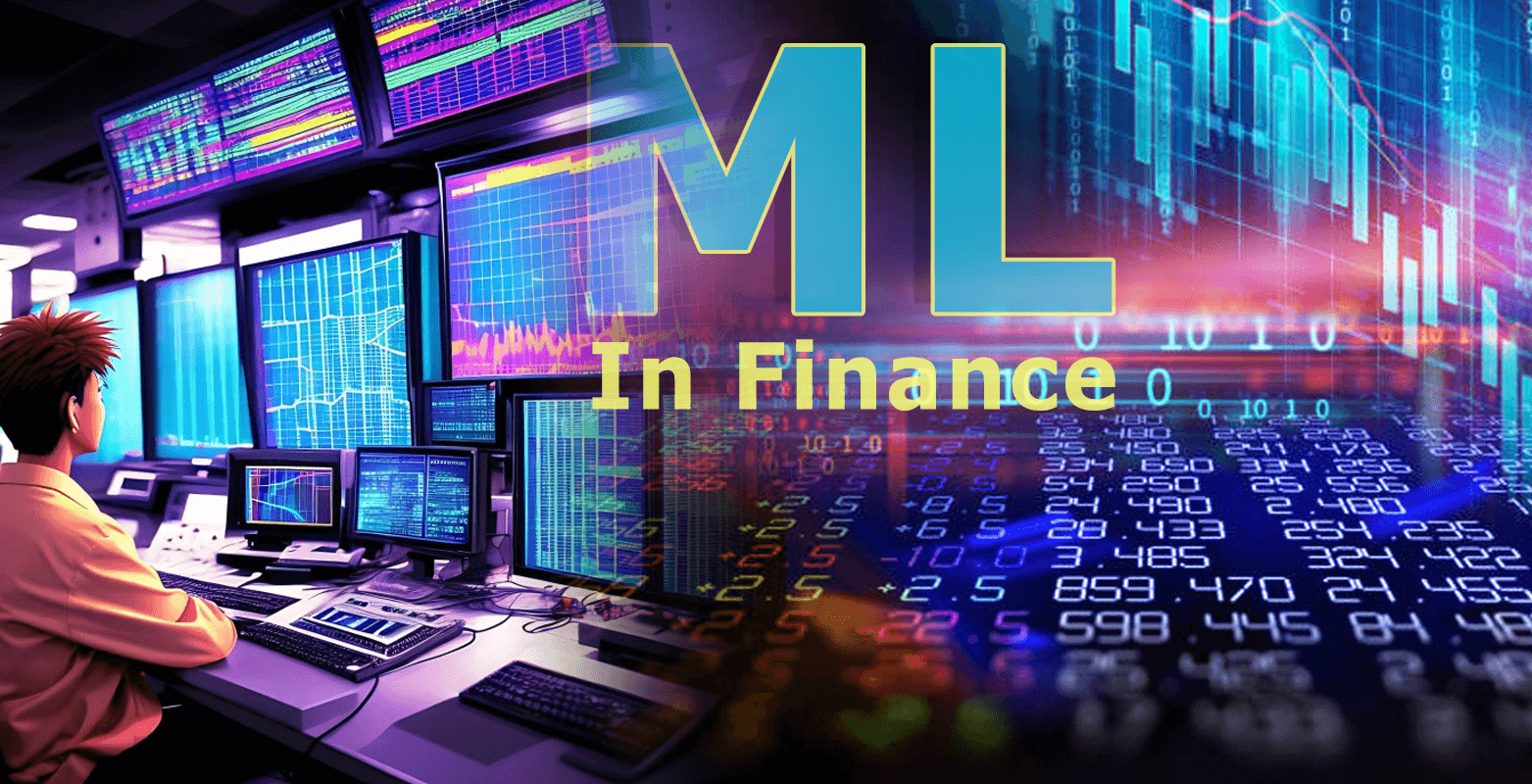
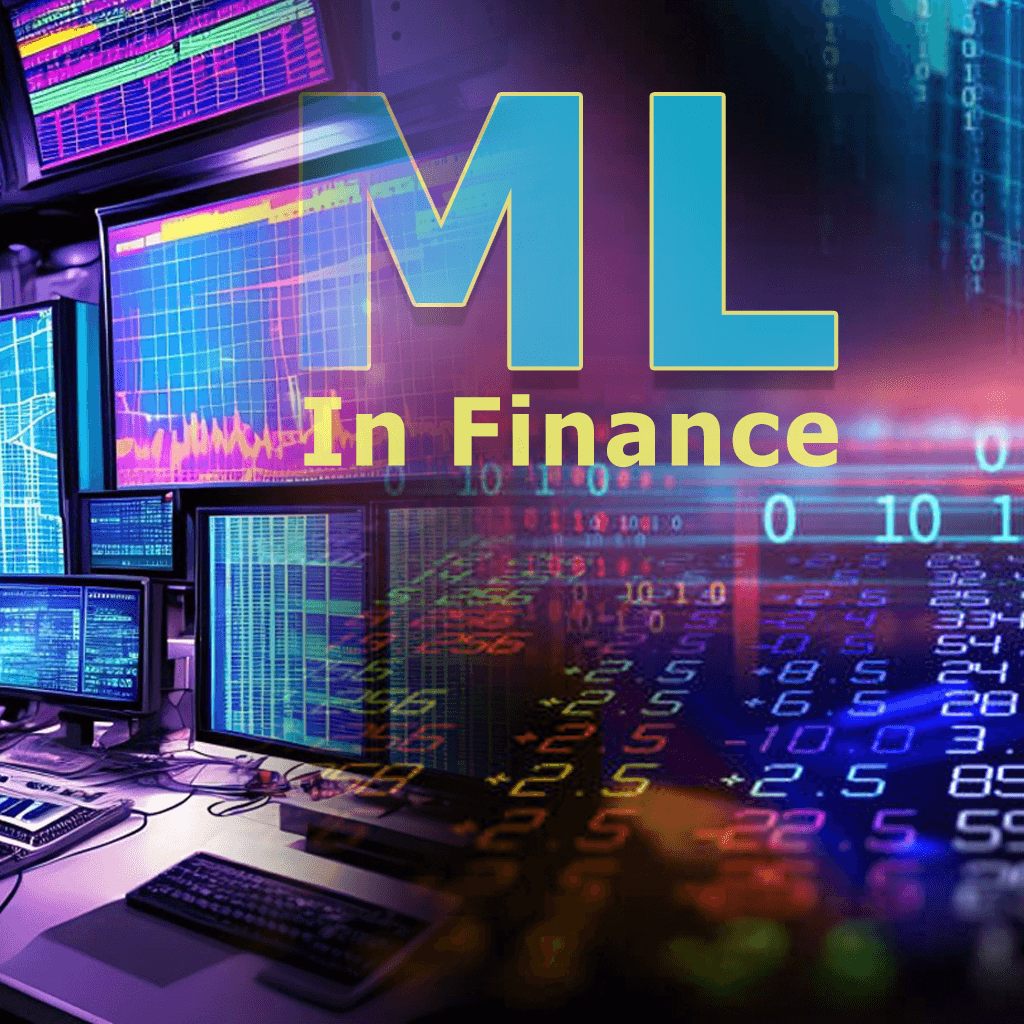
Introduction
In the ever-evolving panorama of finance, gadgets gaining knowledge of (ML) have emerged as a formidable pressure, promising to reshape the industry's dynamics. Its capability to sift through tremendous datasets and determine styles presents opportunities for revolutionary adjustments in decision-making tactics. However, with innovation comes inherent risks and challenges. This blog post delves into the technical intricacies of machine learning in finance, examining whether it stands as a game-changer or introduces potential risk factors.
The Power of Machine Learning in Finance:
Machine learning algorithms have ushered in a new era of data processing in real-time, empowering financial institutions to make more informed and strategic decisions. This transformative technology excels in identifying patterns, correlations, and anomalies that may elude human analysts. Its applications span diverse areas, from predicting stock prices and detecting fraudulent transactions to assessing credit risk and optimising trading strategies.
A pivotal advantage of machine learning in finance lies in its adaptive nature. These algorithms continuously learn and refine their models as new data becomes available, ensuring that financial institutions remain agile and well-informed in a dynamic market. The continuous learning process enhances predictive accuracy and positions financial professionals to make optimal investment decisions.
Risk Factors and Challenges:
Despite the tremendous potential, machine learning in finance comes with its set of risks and challenges that demand careful consideration.
Quality and Availability of Data:
One significant challenge revolves around the quality and availability of data. Machine learning algorithms heavily rely on high-quality, relevant data to make accurate predictions. In the financial realm, data can often be noisy, incomplete, and susceptible to biases. Therefore, ensuring data quality and suitability is paramount for the success of machine learning applications in finance.
Interpretability of Models:
Unlike traditional statistical models that provide transparent explanations for their predictions, machine learning models often operate as "black boxes." This lack of interpretability raises concerns about transparency and accountability, essential elements for regulatory compliance and effective risk management in financial institutions.
Adaptability to Changing Market Conditions:
Relying on historical data poses challenges in swiftly changing market conditions. Financial markets are influenced by various factors, such as economic events, political developments, and market sentiment. Machine learning models trained on historical data may struggle to adapt to sudden shifts, potentially leading to inaccurate predictions and suboptimal decisions.
Addressing the Risks: Education and Expertise:
To unlock the ability of machine learning in finance and mitigate associated risks, financial professionals need to equip themselves with vital schooling and expertise. Institutions just like Indian Institute of Quantitative Finance (IIQF®️) offer specialised programs tailored to bridge the distance between finance and machine learning.
Taking a course at IIQF®️ provides professionals with a robust foundation in both finance and machine learning. Participants gain insights into various machine learning algorithms, data preprocessing techniques, model evaluation, and risk management strategies. These courses also emphasise ethical considerations and regulatory frameworks, ensuring professionals are well-prepared to navigate the challenges associated with machine learning in finance.
Conclusion:
Machine learning holds the promise of revolutionising the finance industry, offering more accurate predictions, improved risk assessment, and enhanced decision-making capabilities. Yet, it is imperative to acknowledge and address the risks that accompany this transformative technology. Financial institutions must prioritise data quality, tackle model interpretability issues, and remain agile in adapting to changing market conditions.
Investing in education and expertise becomes the cornerstone for effectively leveraging the power of machine learning while navigating associated risks. Institutions like IIQF®️ play a crucial role in providing courses that furnish individuals with the knowledge and skills needed to thrive at the intersection of finance and machine learning. With the right approach, machine learning can indeed be a game-changer in finance, transforming the way professionals understand and manage the complexities of the financial world.